Speech
The mission of the Seed Speech team is to enrich interactive and creative processes through the application of multimodal speech technologies. The team focuses on the forefront of research and product development in speech and audio, music, natural language understanding, and multimodal deep learning.
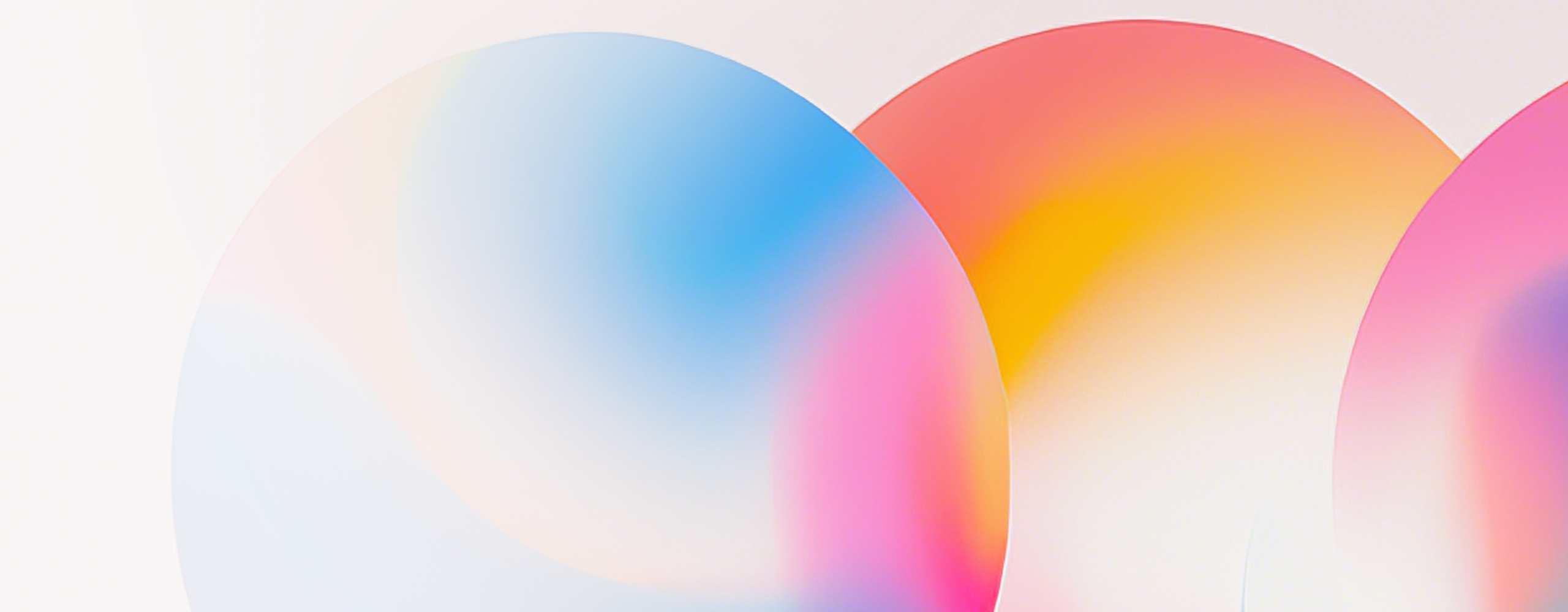
Main areas of focus
AI system technology
We develop AI training and inference systems utilizing GPU technology. We are dedicated to advancing the forefront of AI system technology to accelerate the large language models for audio/music.
AI foundation models for speech/multimodal audio/music
The team is also responsible for developing AI foundation models for speech, multimodal audio, and music throughout the engineering cycle. This includes supporting tasks such as data preparation and processing, model training, evaluation and deployment, among others.
Research topics
Work alongside well-known experts in the voice industry to explore the most challenging research topics. Strive for innovation and set your sights on the pinnacle of excellence in your work, while embarking on a journey of extraordinary personal and professional growth.
Research topics
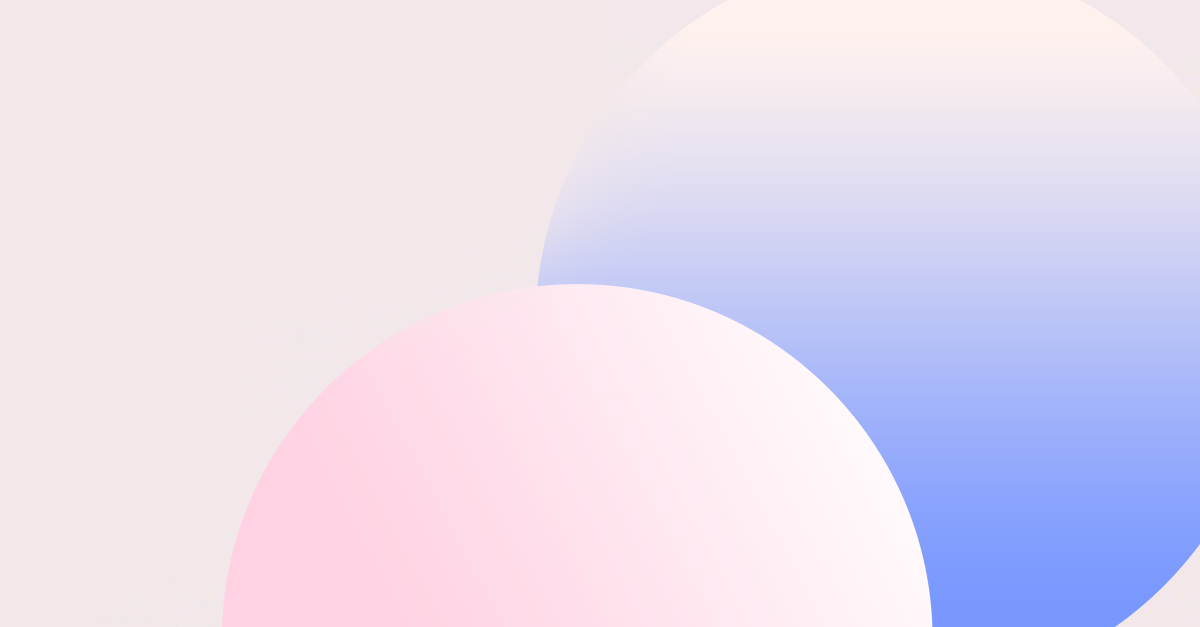
AI foundation models for audio understanding and generation
AI foundation models for audio understanding and generation, exploring standardized modeling approaches for speech recognition, synthesis, transformation, music creation, and sound effect production.
AI foundation
Audio
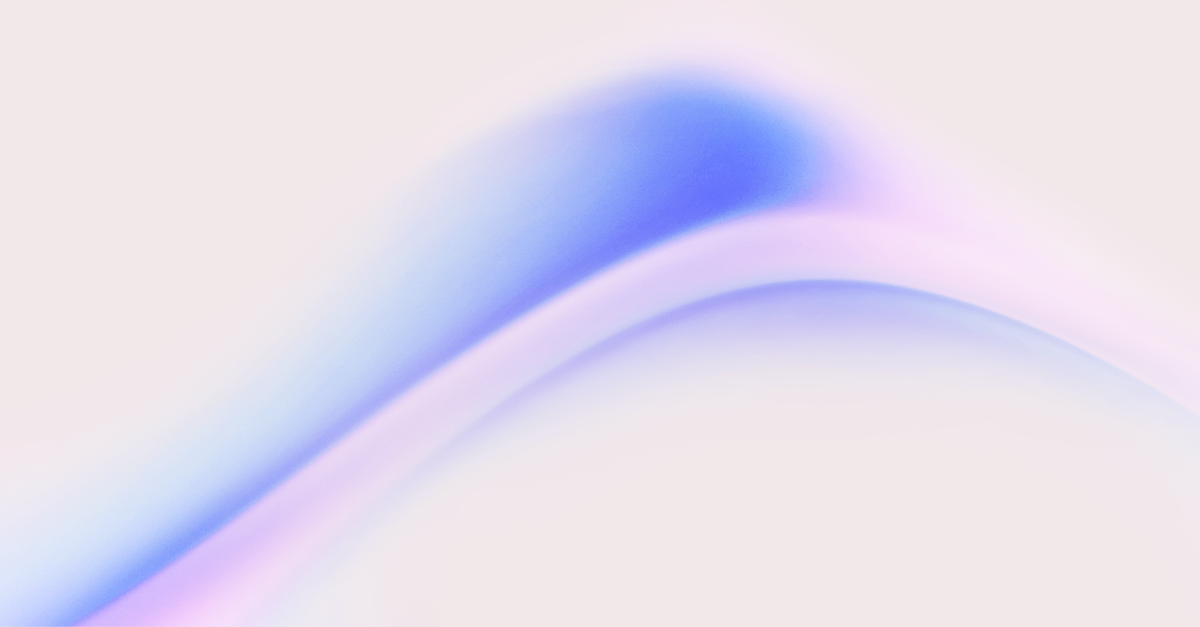
Multimodal model design and optimization
Multimodal model network architecture design and optimization, the design and refinement of diffusion models.
Multimodal
Optimization
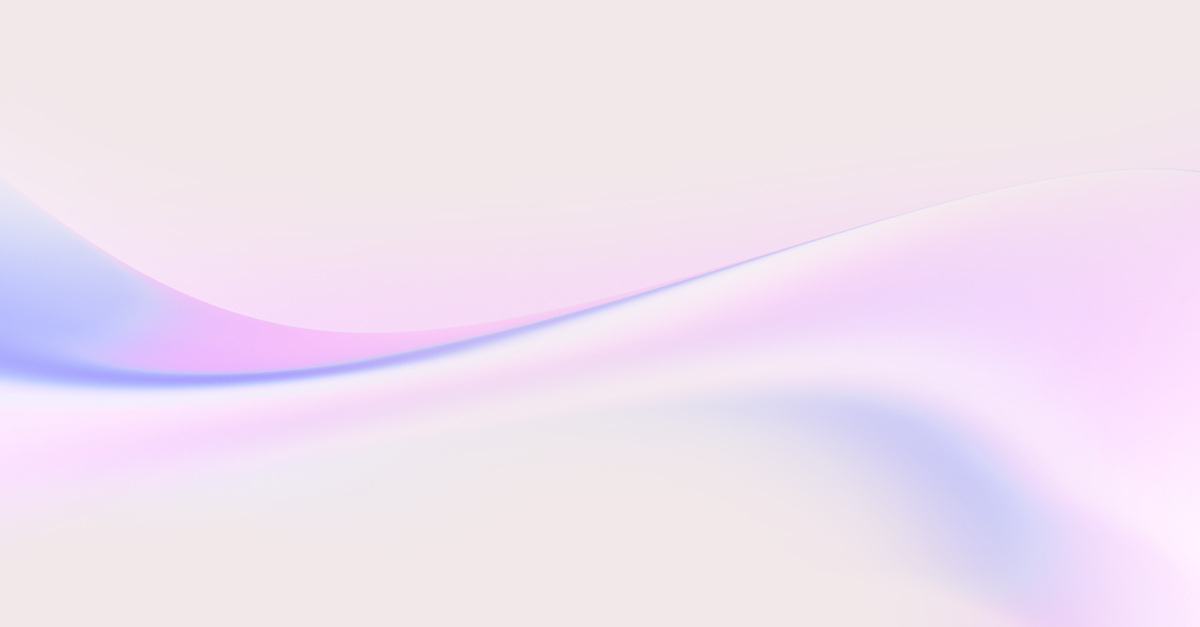
Application of reinforcement learning within environments for audio
Application of reinforcement learning within multimodal large model environments for speech/audio, and the design and enhancement of RL system solutions.
Reinforcement learning
Application
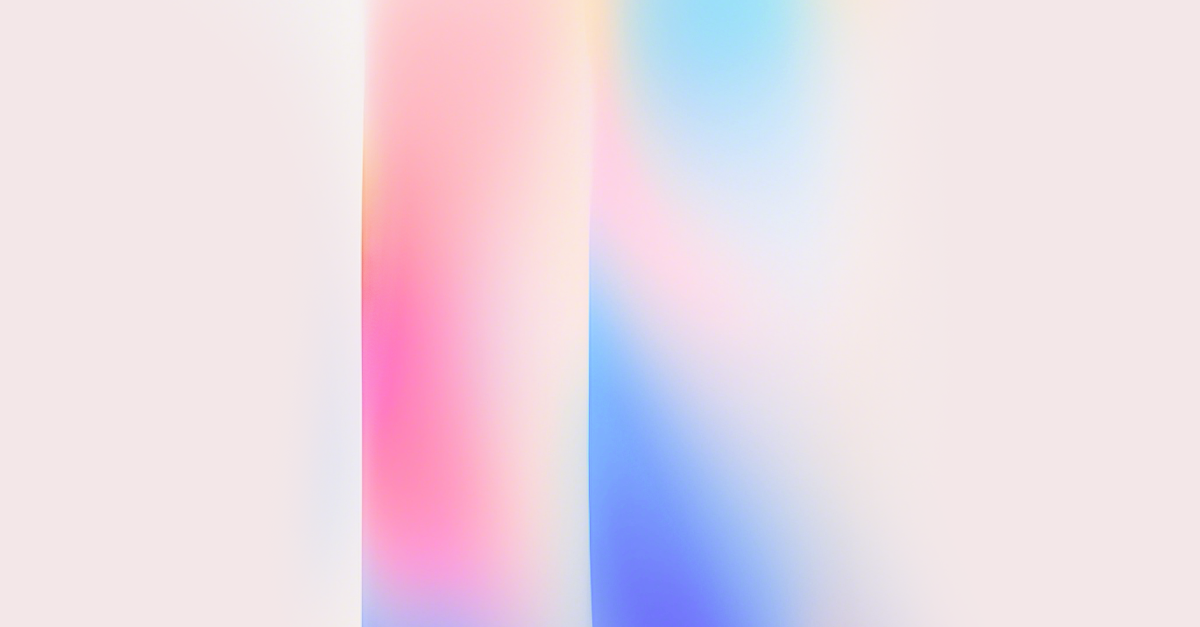
Large-scale distributed training and inference systems
Explore the development of efficient, large-scale distributed training and inference systems.
Large-scale
System
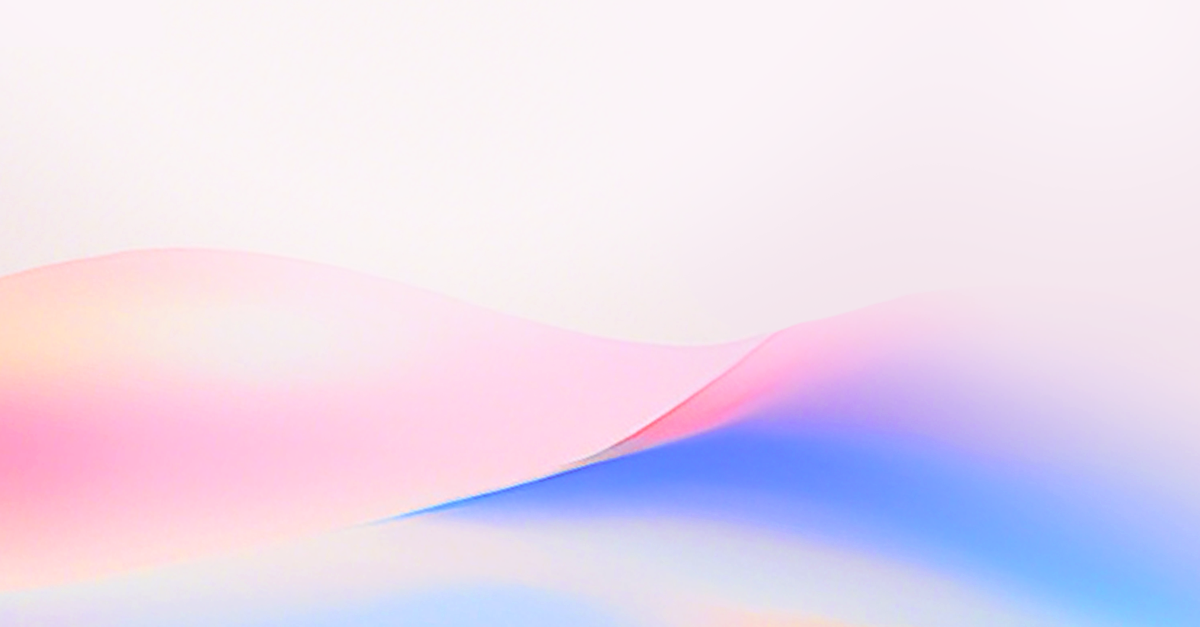
Development of machine learning platforms within environments for speech
Development of robust, scalable, and distributed machine learning platforms, aimed at facilitating the production and rapid iteration of speech/audio-related algorithms.
Machine learning
Audio
Selected Papers
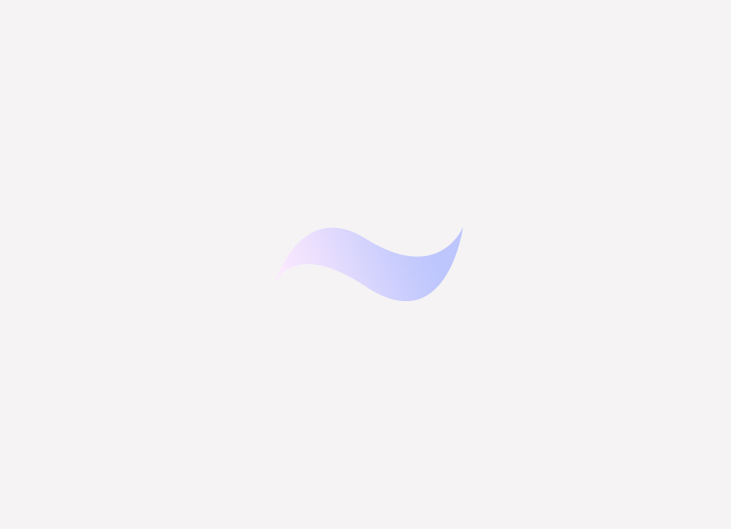
Feb 25, 2025
You Only Sample Once: Taming One-Step Text-to-Image Synthesis by Self-Cooperative Diffusion GANs
Recently, some works have tried to combine diffusion and Generative Adversarial Networks (GANs) to alleviate the computational cost of the iterative denoising inference in Diffusion Models (DMs). However, existing works in this line suffer from either training instability and mode collapse or subpar one-step generation learning efficiency. To address these issues, we introduce YOSO, a novel generative model designed for rapid, scalable, and high-fidelity one-step image synthesis with high training stability and mode coverage. Specifically, we smooth the adversarial divergence by the denoising generator itself, performing self-cooperative learning. We show that our method can serve as a one-step generation model training from scratch with competitive performance. Moreover, we extend our YOSO to one-step text-to-image generation based on pre-trained models by several effective training techniques (i.e., latent perceptual loss and latent discriminator for efficient training along with the latent DMs; the informative prior initialization (IPI), and the quick adaption stage for fixing the flawed noise scheduler). Experimental results show that YOSO achieves the state-of-the-art one-step generation performance even with Low-Rank Adaptation (LoRA) fine-tuning. In particular, we show that the YOSO-PixArt-α can generate images in one step trained on 512 resolution, with the capability of adapting to 1024 resolution without extra explicit training, requiring only ~10 A800 days for fine-tuning. Our code is provided at this https URL[https://github.com/Luo-Yihong/YOSO].
Yihong Luo, Xiaolong Chen, Xinghua Qu, Tianyang Hu, Jing Tang
Computer Vision
2025.02.25
You Only Sample Once: Taming One-Step Text-to-Image Synthesis by Self-Cooperative Diffusion GANs
Yihong Luo, Xiaolong Chen, Xinghua Qu, Tianyang Hu, Jing Tang
Speech
Computer Vision
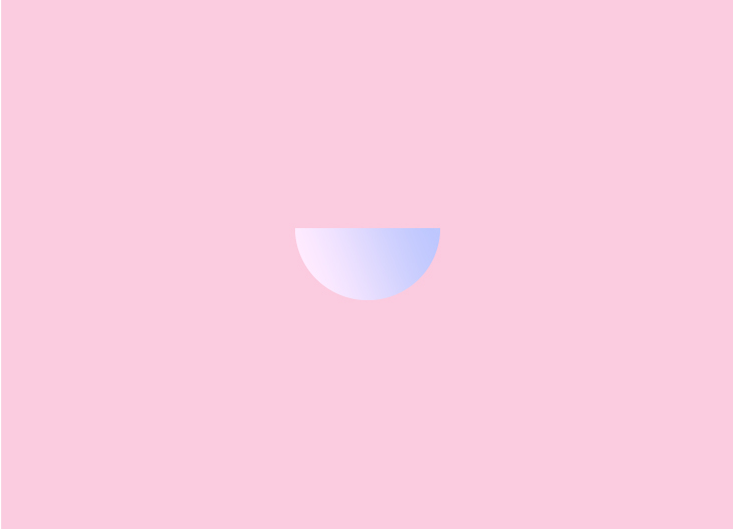
Sep 13, 2024
Seed-Music: A Unified Framework for High Quality and Controlled Music Generation
We introduce Seed-Music, a suite of music generation systems capable of producing high-quality music with fine-grained style control. Our unified framework leverages both auto-regressive language modeling and diffusion approaches to support two key music creation workflows: controlled music generation and postproduction editing. For controlled music generation, our system enables vocal music generation with performance controls from multi-modal inputs, including style descriptions, audio references, musical scores, and voice prompts. For postproduction editing, it offers interactive tools for editing lyrics and vocal melodies directly in the generated audio.
We encourage readers to listen to demo audio examples at https://team.doubao.com/seed-music.
Ye Bai, Haonan Chen, Jitong Chen, Zhuo Chen, Yi Deng, Xiaohong Dong, Lamtharn Hantrakul, Weituo Hao, Qingqing Huang, Zhongyi Huang, Dongya Jia, Feihu La, Duc Le, Bochen Li, Chumin Li, Hui Li, Xingxing Li, Shouda Liu, Wei-Tsung Lu, Yiqing Lu, Andrew Shaw, Janne Spijkervet, Yakun Sun, Bo Wang, Ju-Chiang Wang, Yuping Wang, Yuxuan Wang, Ling Xu, Yifeng Yang, Chao Yao, Shuo Zhang, Yang Zhang, Yilin Zhang, Hang Zhao, Ziyi Zhao, Dejian Zhong, Shicen Zhou, Pei Zou
Speech&Audio
2024.09.13
Seed-Music: A Unified Framework for High Quality and Controlled Music Generation
Ye Bai, Haonan Chen, Jitong Chen, Zhuo Chen, Yi Deng, Xiaohong Dong, Lamtharn Hantrakul, Weituo Hao, Qingqing Huang, Zhongyi Huang, Dongya Jia, Feihu La, Duc Le, Bochen Li, Chumin Li, Hui Li, Xingxing Li, Shouda Liu, Wei-Tsung Lu, Yiqing Lu, Andrew Shaw, Janne Spijkervet, Yakun Sun, Bo Wang, Ju-Chiang Wang, Yuping Wang, Yuxuan Wang, Ling Xu, Yifeng Yang, Chao Yao, Shuo Zhang, Yang Zhang, Yilin Zhang, Hang Zhao, Ziyi Zhao, Dejian Zhong, Shicen Zhou, Pei Zou
Speech
Speech&Audio
Learn More
Technical capability demonstration
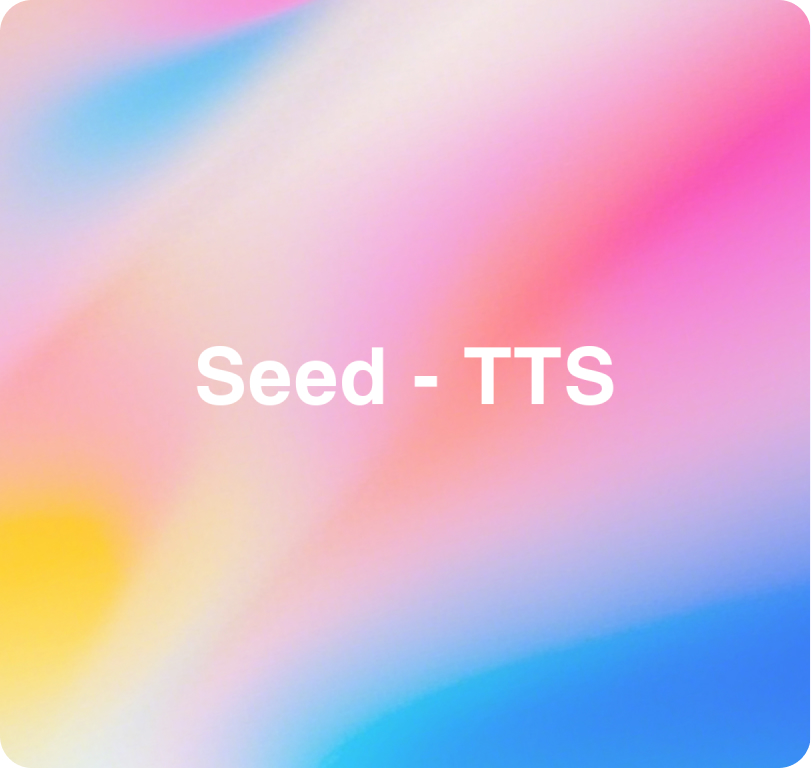
Seed-TTS
Doubao · Speech Generation Model shows superior context comprehension and naturalness, capable of deeply understanding narratives and character roles, expressing emotions accurately, and maintaining specific pronunciation habits such as swallowing sounds and accents, closely mimicking human vocal quality.
Speech
Generation
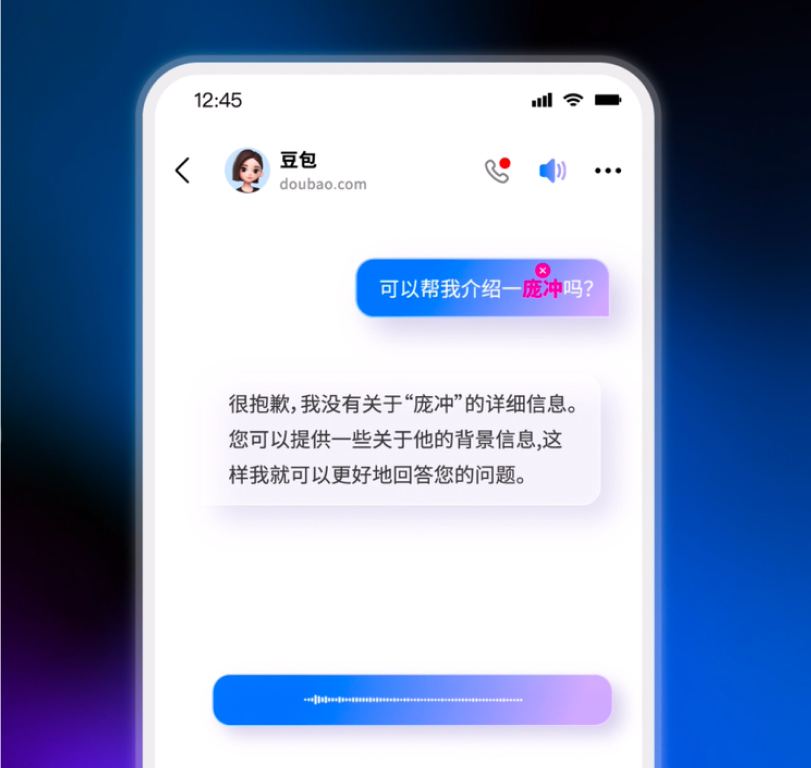
Seed-ASR
Doubao · Speech Recognition Model leverages more advanced context-aware capabilities to produce more precise recognition outcomes and support the recognition of multiple Chinese dialects, including Mandarin, Cantonese, Shanghainese, Sichuanese, Xi'an dialect, and Minnan, within a single model.
Speech
Recognition
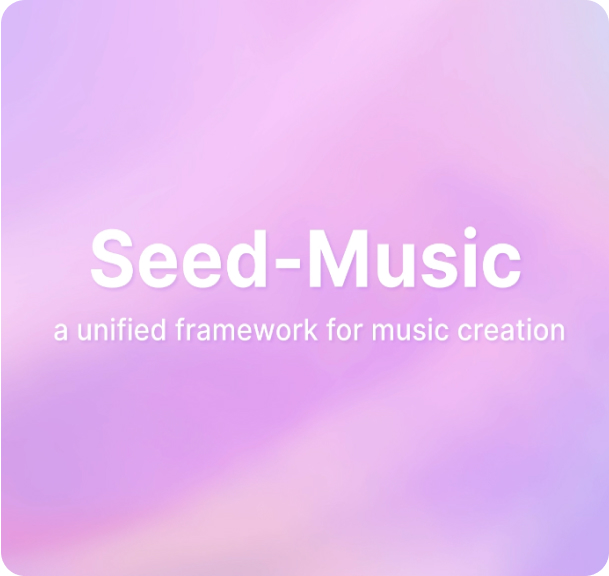
Seed-Music
Seed-Music is a collection of music generation models with flexible control capabilities, offering four core functions: controllable music generation, score-to-music conversion, lyric and music editing, and zero-sample vocal cloning. By smartly merging the benefits of language and diffusion models into the composition process, Music makes crafting songs accessible to everyone.
Music
Generation
Featured Jobs
Research Scientist, Multimodality
Research Scientist, Foundation Model, Music Intelligence
Research Scientist in Foundation Model, Speech & Audio Generation - 2025 Start (PhD)
Research Scientist in Foundation Model, Music - 2025 Start (PhD)
Student Researcher (Doubao (Seed) - Foundation Model - Speech Understanding) - 2025 Start (PhD)
Student Researcher (Doubao (Seed) - Music Foundation Model) - 2025 Start (PhD)