*01
Seed Edge Program Introduction
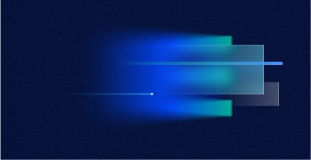
The Seed Edge is a long-term research program under the ByteDance Doubao (Seed) Team dedicated to exploring innovative paths to general intelligence. The program focuses on long-term, aggressive, and potentially uncertain AI research projects and encourages cross-team and cross-modal collaboration. We provide an open research environment, offer dedicated computing resources, and adopt a longer-term performance review cycle for our members.
*02
Research Areas of Seed Edge
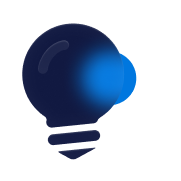
Exploring the Boundaries of Reasoning Abilities
The technical approach featuring O1 demonstrates that reasoning capabilities can significantly advance the boundaries of intelligence. However, our practical experience reveals that reasoning research is still in its early stages, with numerous issues to explore. Whether large-scale reinforcement learning with pre-training level computing resources, iteration of pre-training and reinforcement learning, or generalisable test-time scaling, each technological breakthrough pushes the boundaries of intelligence further.
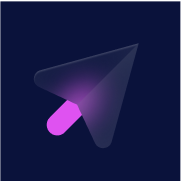
Exploring the Boundaries of Perception Abilities
We believe that intelligence and interaction are interconnected. General models must possess a high level of intelligence and exhibit exceptional capabilities in human communication and interaction. We will unify generation and understanding representations and explore world modelling. We will also compress raw data from the real world and investigate world modelling that surpasses language in representations, thereby breaking free from the language constraints on intelligence boundaries.
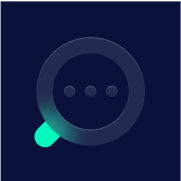
Exploring Next Scaling Direction
Following pretraining and test-time scaling, the next scaling direction will be crucial in evolving intelligence boundaries in the future. We will conduct explorations in the next scaling direction and push the boundaries of intelligence.
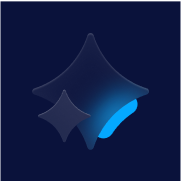
Exploring Next-Generation Learning Paradigms
We aim to explore areas of change in future learning paradigms that challenge the "consensus" of existing ones, thus providing a foundation and possibilities for achieving general intelligence efficiently. We intend to understand the origins of model learning capabilities through explainability research. This will inform novel learning perspectives that allow us to explore model performance in the learning process and compare it to biologically inspired learning processes. It will also identify areas for improvement in existing model learning paradigms, such as exploring more efficient learning objectives than next-token prediction, more efficient learning methods than back-propagation, and more efficient learning paradigms than large-scale pre-training and alignment.
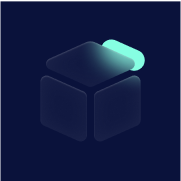
Exploring Next-Generation Model Design Integrating Software and Hardware
Transformers' efficiency is primarily determined by their compatibility with GPUs, allowing for the full GPU performance. We aim to design next-generation models tailored to the architectural characteristics of next-generation training and inference hardware. By adopting a holistic approach integrating both software and hardware, we will optimise training efficiency, inference efficiency, and model performance in parallel, maximising the capabilities of next-generation hardware and pushing the boundaries of model capabilities.
*03
Here At Seed Edge, You Can Enjoy
- Free and Open Research EnvironmentThe Seed Edge program is dedicated to finding new paths to general intelligence. We encourage our members to propose new research questions and form flexible project teams to explore them.
- Independently-Managed Computational ResourcesThe Seed Edge Research Program independently manages and allocates all necessary computational resources based on research projects.
- Commitment to Long-Term ResearchWe extend the review cycle for Seed Edge members, highlighting the value and impact of long-term research outcomes.
- Encouragement of Cross-Modal CollaborationWe encourage cross-team and cross-modal communication and collaboration to promote the interconnection and integration of cross-modal knowledge.
*01
Seed Edge Program Introduction
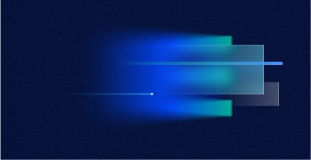
The Seed Edge is a long-term research program under the ByteDance Doubao (Seed) Team dedicated to exploring innovative paths to general intelligence. The program focuses on long-term, aggressive, and potentially uncertain AI research projects and encourages cross-team and cross-modal collaboration. We provide an open research environment, offer dedicated computing resources, and adopt a longer-term performance review cycle for our members.
*02
Research Areas of Seed Edge
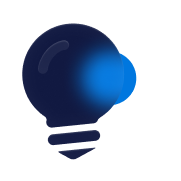
Exploring the Boundaries of Reasoning Abilities
The technical approach featuring O1 demonstrates that reasoning capabilities can significantly advance the boundaries of intelligence. However, our practical experience reveals that reasoning research is still in its early stages, with numerous issues to explore. Whether large-scale reinforcement learning with pre-training level computing resources, iteration of pre-training and reinforcement learning, or generalisable test-time scaling, each technological breakthrough pushes the boundaries of intelligence further.
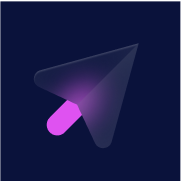
Exploring the Boundaries of Perception Abilities
We believe that intelligence and interaction are interconnected. General models must possess a high level of intelligence and exhibit exceptional capabilities in human communication and interaction. We will unify generation and understanding representations and explore world modelling. We will also compress raw data from the real world and investigate world modelling that surpasses language in representations, thereby breaking free from the language constraints on intelligence boundaries.
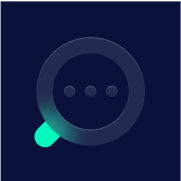
Exploring Next Scaling Direction
Following pretraining and test-time scaling, the next scaling direction will be crucial in evolving intelligence boundaries in the future. We will conduct explorations in the next scaling direction and push the boundaries of intelligence.
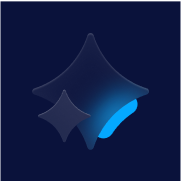
Exploring Next-Generation Learning Paradigms
We aim to explore areas of change in future learning paradigms that challenge the "consensus" of existing ones, thus providing a foundation and possibilities for achieving general intelligence efficiently. We intend to understand the origins of model learning capabilities through explainability research. This will inform novel learning perspectives that allow us to explore model performance in the learning process and compare it to biologically inspired learning processes. It will also identify areas for improvement in existing model learning paradigms, such as exploring more efficient learning objectives than next-token prediction, more efficient learning methods than back-propagation, and more efficient learning paradigms than large-scale pre-training and alignment.
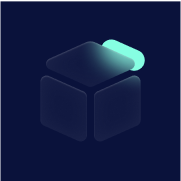
Exploring Next-Generation Model Design Integrating Software and Hardware
Transformers' efficiency is primarily determined by their compatibility with GPUs, allowing for the full GPU performance. We aim to design next-generation models tailored to the architectural characteristics of next-generation training and inference hardware. By adopting a holistic approach integrating both software and hardware, we will optimise training efficiency, inference efficiency, and model performance in parallel, maximising the capabilities of next-generation hardware and pushing the boundaries of model capabilities.
*03
Here At Seed Edge, You Can Enjoy
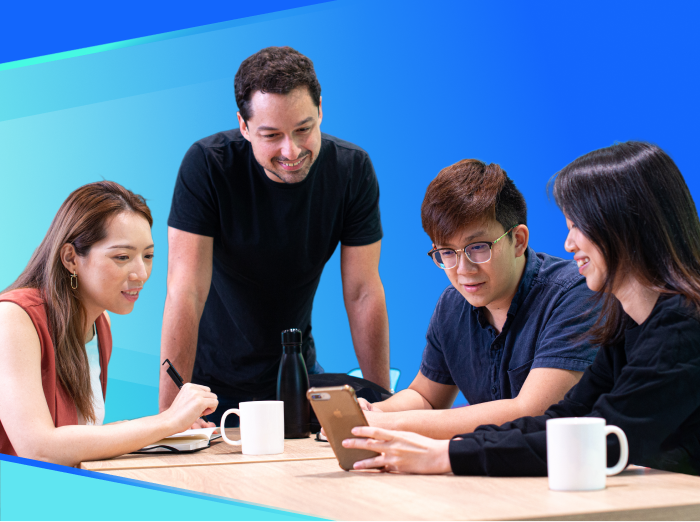
- Free and Open Research EnvironmentThe Seed Edge program is dedicated to finding new paths to general intelligence. We encourage our members to propose new research questions and form flexible project teams to explore them.
- Independently-Managed Computational ResourcesThe Seed Edge Research Program independently manages and allocates all necessary computational resources based on research projects.
- Commitment to Long-Term ResearchWe extend the review cycle for Seed Edge members, highlighting the value and impact of long-term research outcomes.
- Encouragement of Cross-Modal CollaborationWe encourage cross-team and cross-modal communication and collaboration to promote the interconnection and integration of cross-modal knowledge.