Foundation
The Doubao Foundation Model team oversees the engineering framework, architectural design, and code generation for AI foundation models.
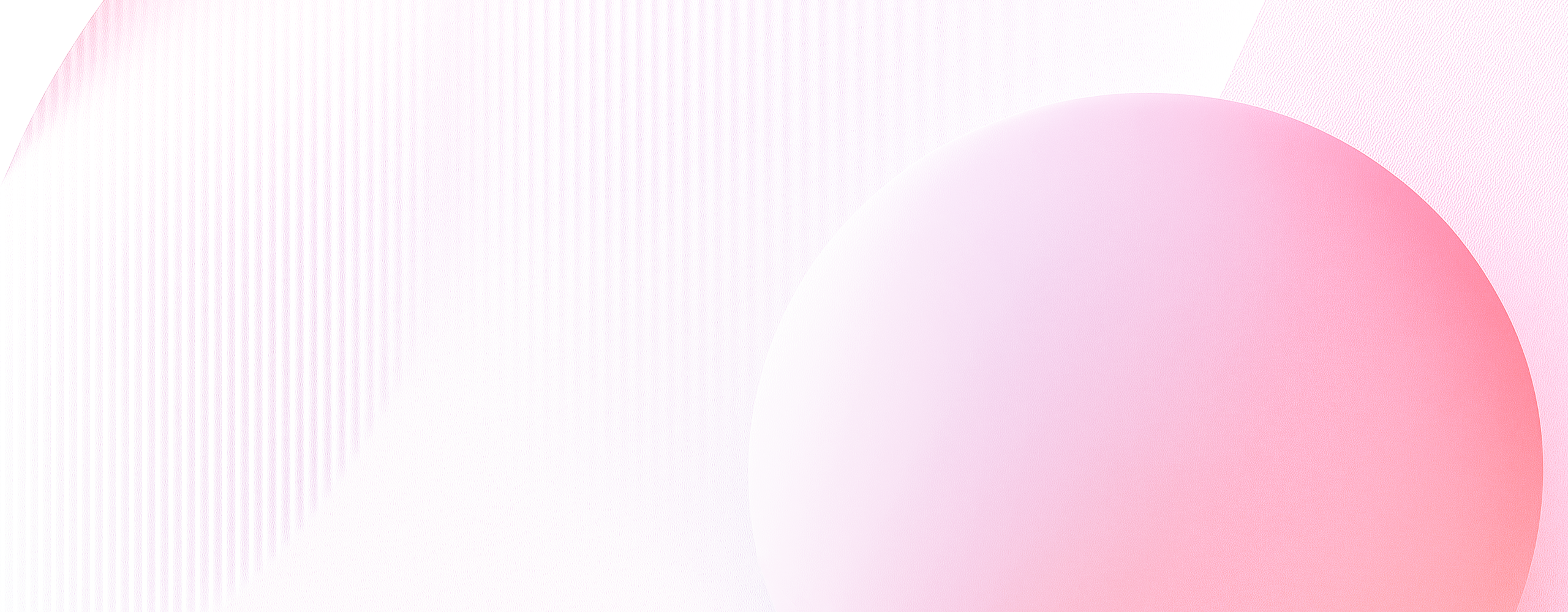
Main areas of focus
Engineering architecture
The tasks involve large-scale distributed training, high-performance inference, and integration with new hardware.
Model structure design
The focus is on training efficient models at a lower cost. This involves exploring sparse models like MoE and a more efficient Attention structure, while collaborating closely with the engineering team to optimize performance.
Code generation
The team focuses on improving the effectiveness of model codes from pre-training to reinforcement learning.
Research topics
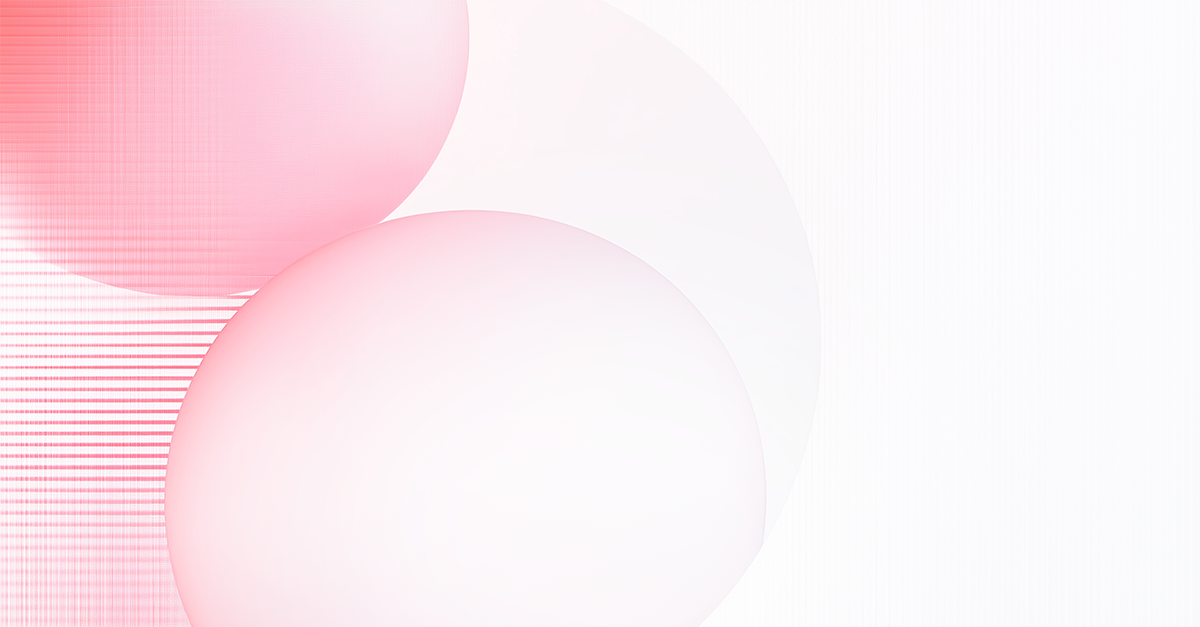
AI foundation model architecture
Design efficient AI foundation model structures to achieve better results with minimal training and inference costs.
AI foundation model
Efficient
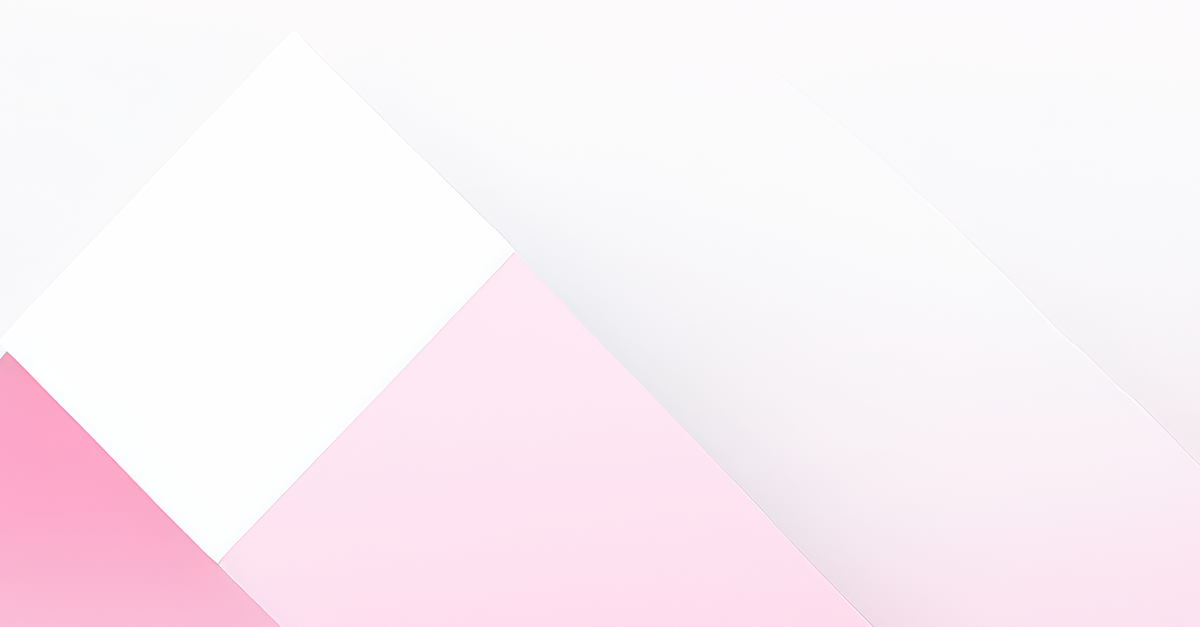
Large-scale training clusters
Study methods to improve the stability and Maximum Throughput Utilization (MFU) of super-large training clusters, including cross-cluster training techniques.
Large-scale
Stability
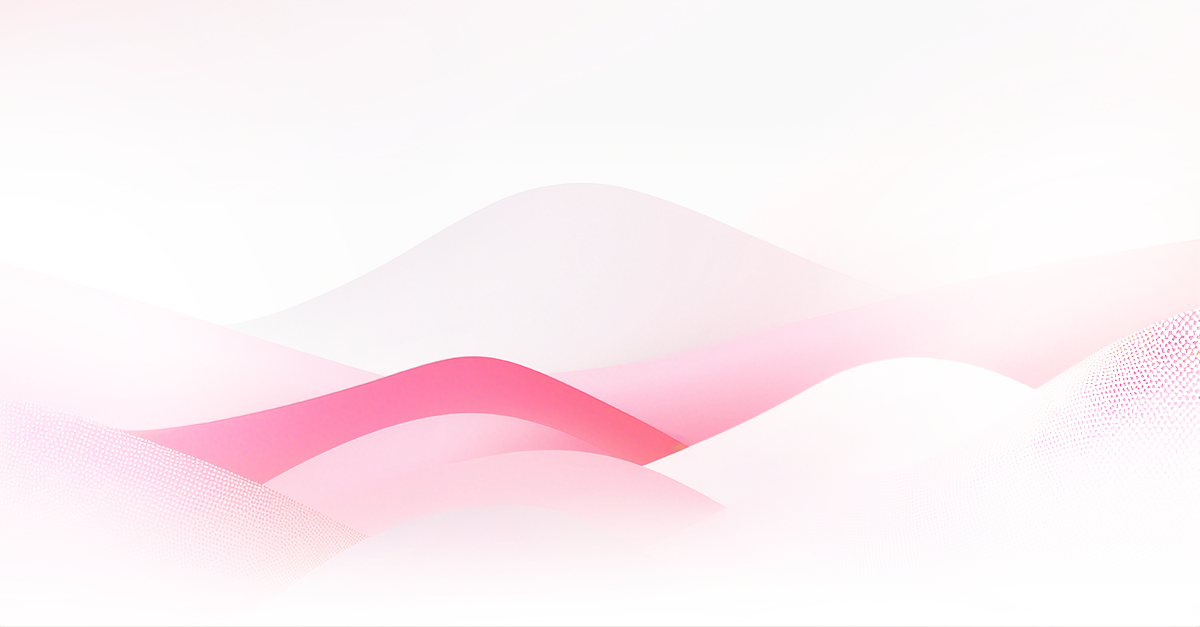
Inference parallelization solutions
Research solutions for memory access limitations during inference, focusing on multi-machine inference and various parallel inference schemes.
Inference
Parallel
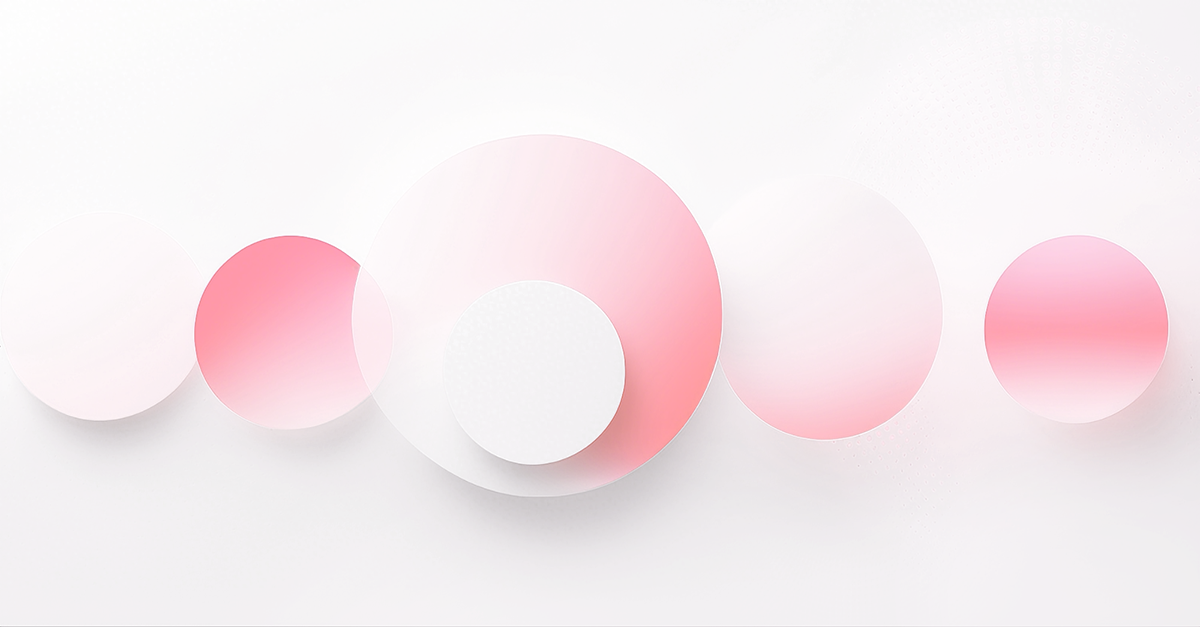
Advanced structures and patterns
Explore the integration of next-generation computing systems to investigate advanced model structures, training methodologies, and inference techniques.
Computing systems
Advanced
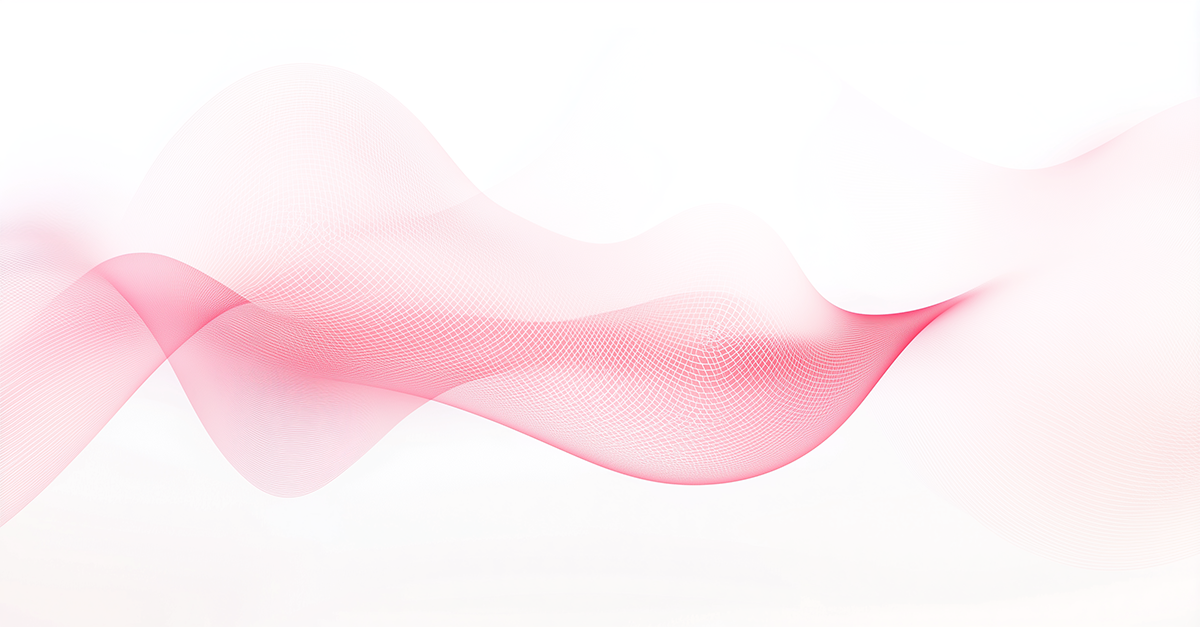
Algorithm innovation
Investigate foundational algorithmic challenges in AI foundation models and identify opportunities for algorithmic innovation.
Algorithm
Innovation
Technical applications
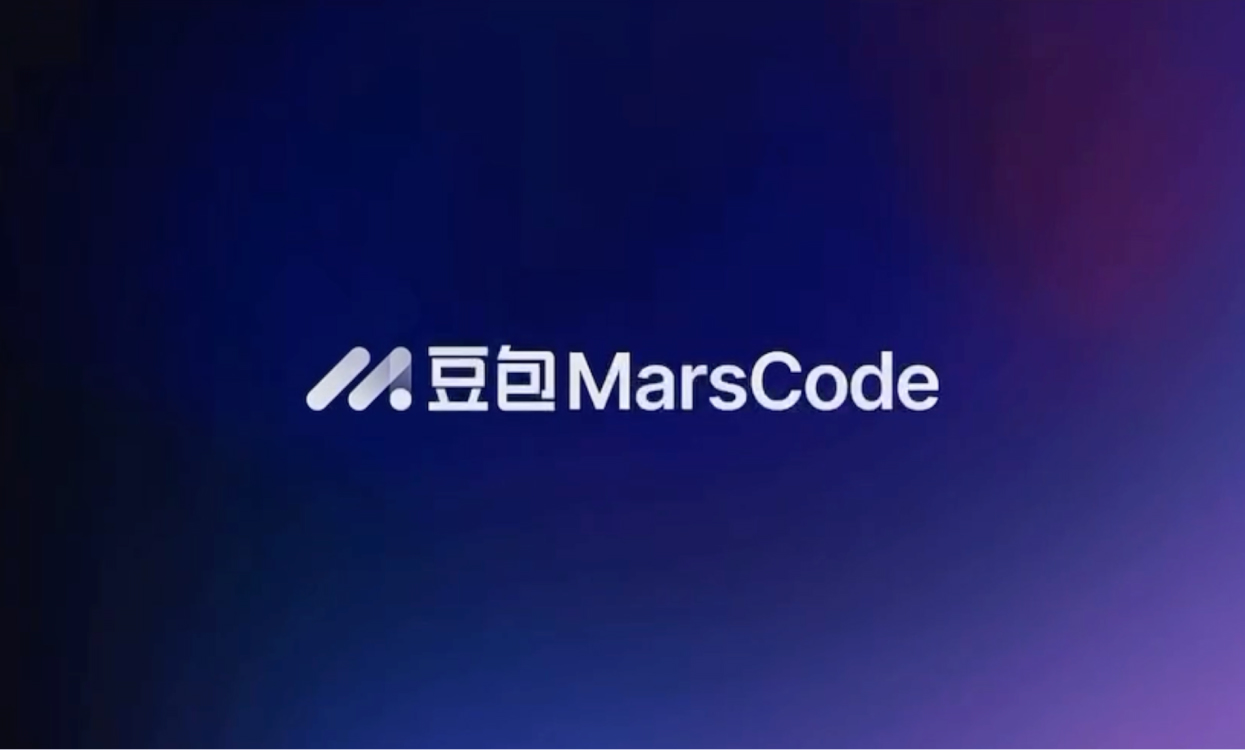
MarsCode
MarsCode is an advanced development tool built on the large-scale Doubao model, offering two primary modes of operation: Cloud IDE and Al Programming Assistant. It provides features such as code completion, intelligent Q&A, code analysis, explanation, and bug fixing, utilizing Al-driven capabilities to boost programming intelligence and reduce development time.
MarsCode intelligently detects the context of ongoing coding tasks and integrates a range of capabilities, including code understanding, generation, optimization, recommendations, completion, and review. These features are seamlessly embedded throughout the R&D process, helping developers improve both the quality and efficiency of their code.
Advanced development
Coding