LLM
The Doubao Large Language Model (LLM) team is dedicated to aggressively advancing the next generation of LLMs, tackling fundamental challenges in LLM development head-on. Our areas of concentration include model self-learning, memory capabilities, long-text generation, and interpretability. We dive deep into the latest technologies and create comprehensive solutions from concept to completion. In our endeavor to adopt LLMs in real-life scenarios, we persistently seek out methods to enhance applications through technological innovation.
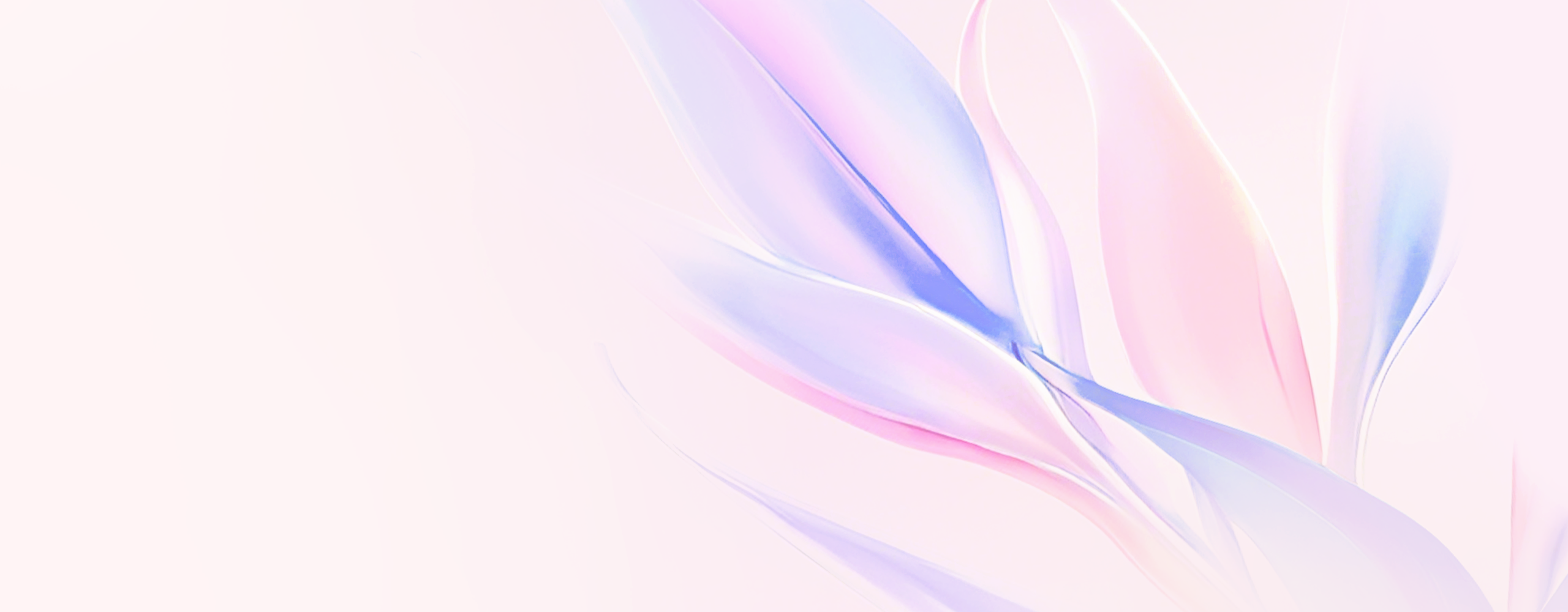
Research topics
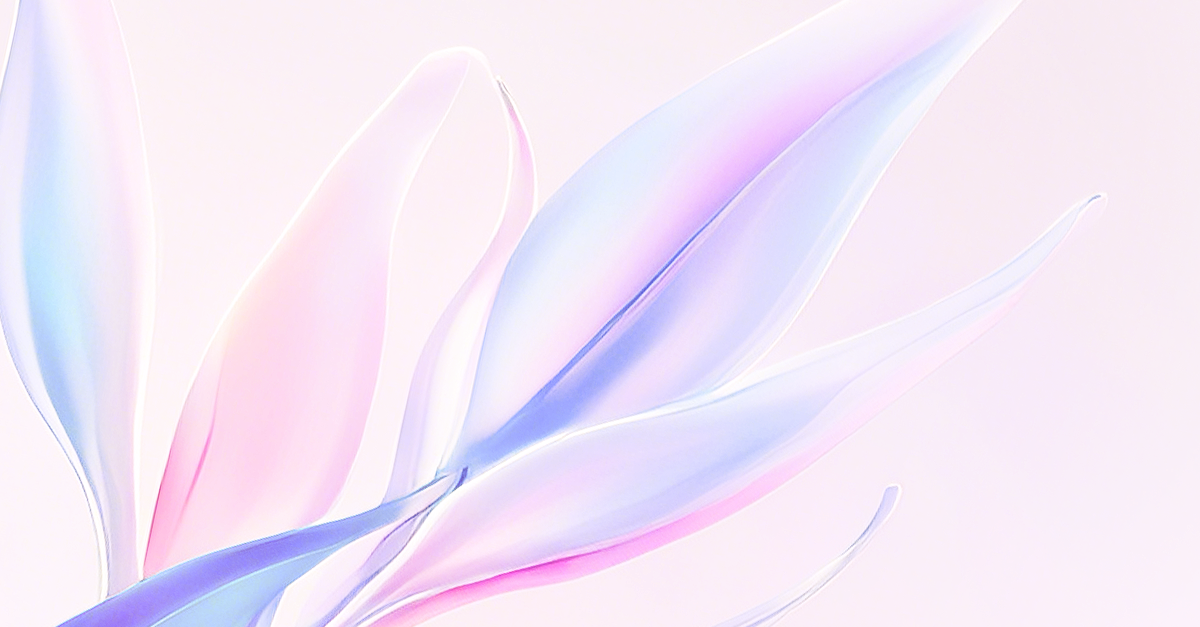
Scalability
Explore more efficient data and modeling techniques during the pre-training stage to ensure that even with a drastic increase in computational power, the Scaling Law remains effectively applicable.
Data
Modeling
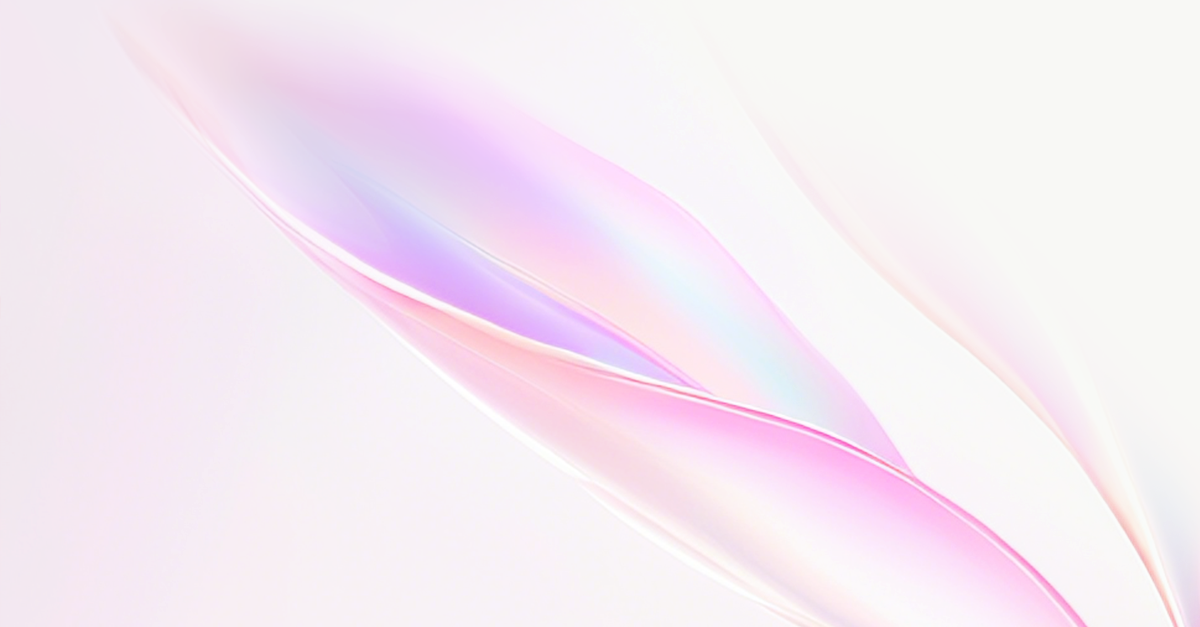
World reward model
Effectively evaluate the quality of responses across various abilities and modalities.
Reward model
Response
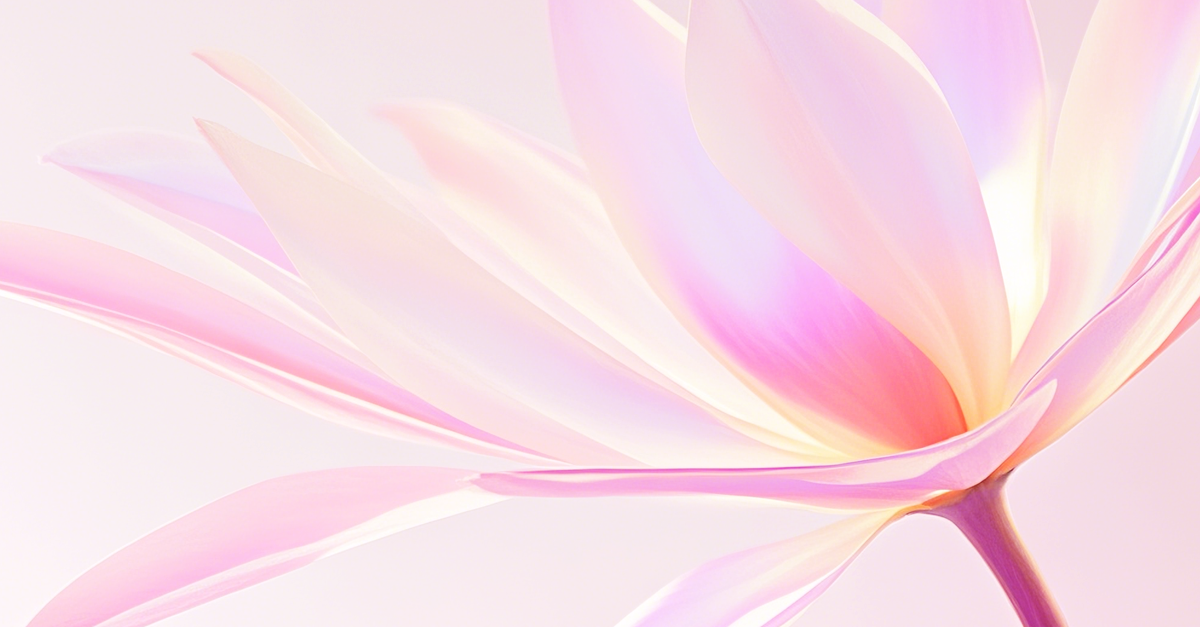
Reinforcement learning generalization and efficiency
This involves exploring innovative reinforcement learning algorithms, integrating and transferring reinforcement learning across different modalities, enhancing exploration and learning efficiency in reinforcement learning algorithms, and generalizing from In-Distribution (IID) to Out-Of-Distribution (OOD).
Generalization
Efficiency
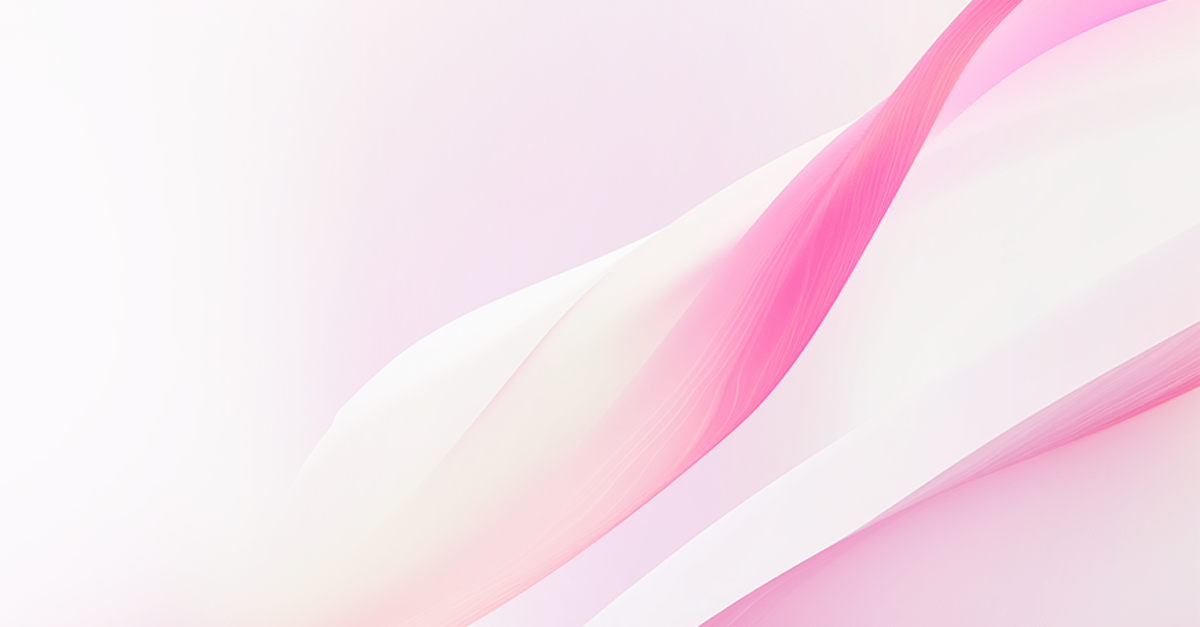
Long-term tasks/planning
Strategies for optimizing reward models and reinforcement learning methods in the context of long-term tasks or planning.
Task
Planning
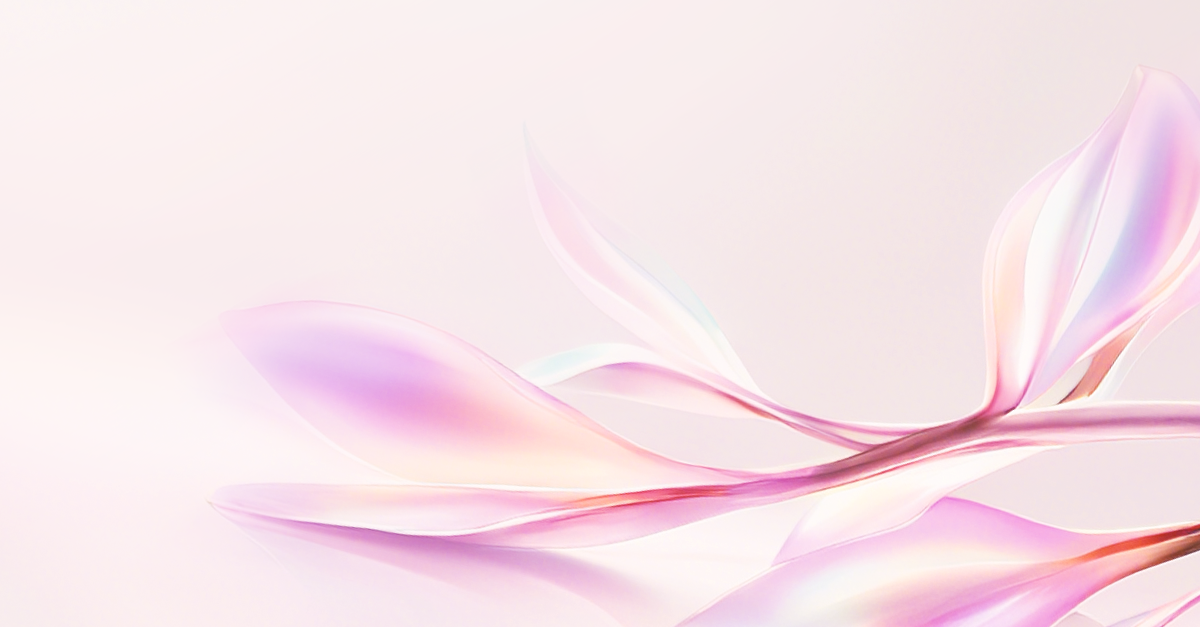
Long memory
Enhance the long-term memory capabilities of models by exploring superior model architectures and data compositions, aiming to improve memory capacity at a manageable cost, thereby enabling bots to serve as personalized assistants for individuals.
Model
Data
View All