Speech
The mission of the Doubao Speech team is to enrich interactive and creative processes through the application of multimodal speech technologies. The team focuses on the forefront of research and product development in speech and audio, music, natural language understanding, and multimodal deep learning.
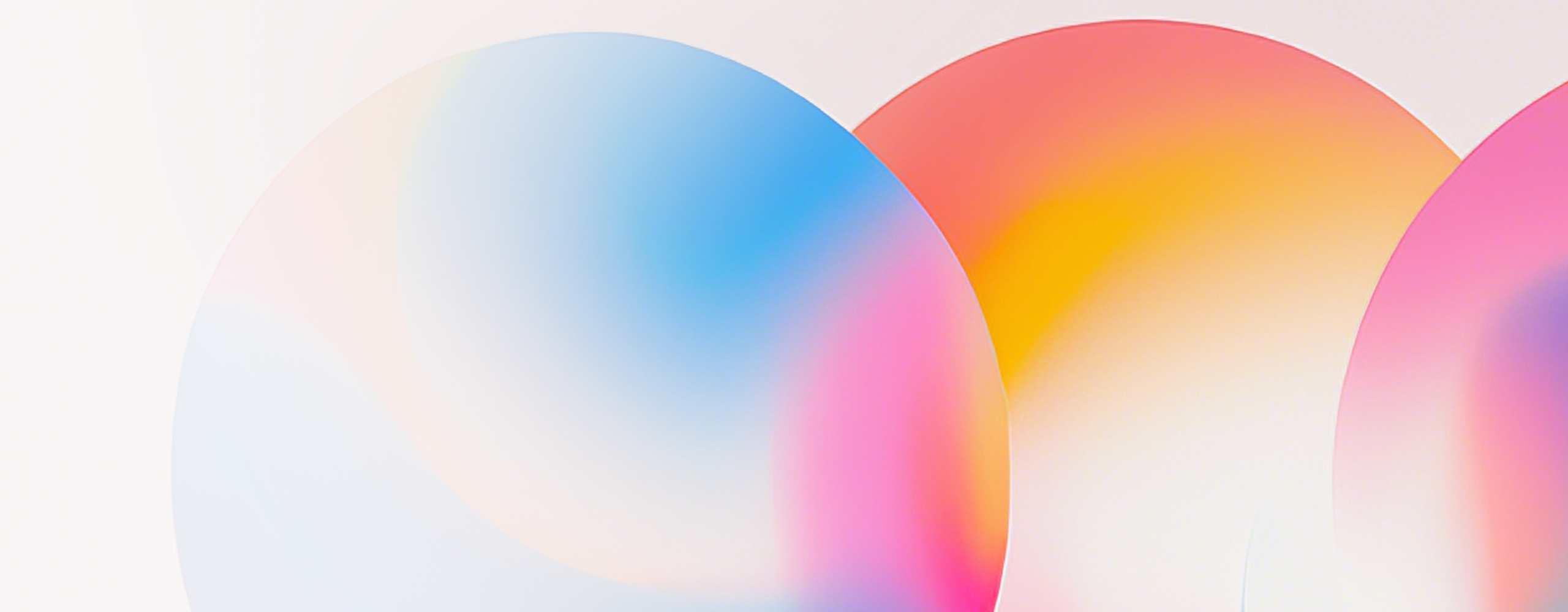
Main areas of focus
AI system technology
We develop AI training and inference systems utilizing GPU technology. We are dedicated to advancing the forefront of AI system technology to accelerate the large language models for audio/music.
AI foundation models for speech/multimodal audio/music
The team is also responsible for developing AI foundation models for speech, multimodal audio, and music throughout the engineering cycle. This includes supporting tasks such as data preparation and processing, model training, evaluation and deployment, among others.
Research topics
Work alongside well-known experts in the voice industry to explore the most challenging research topics. Strive for innovation and set your sights on the pinnacle of excellence in your work, while embarking on a journey of extraordinary personal and professional growth.
Research topics
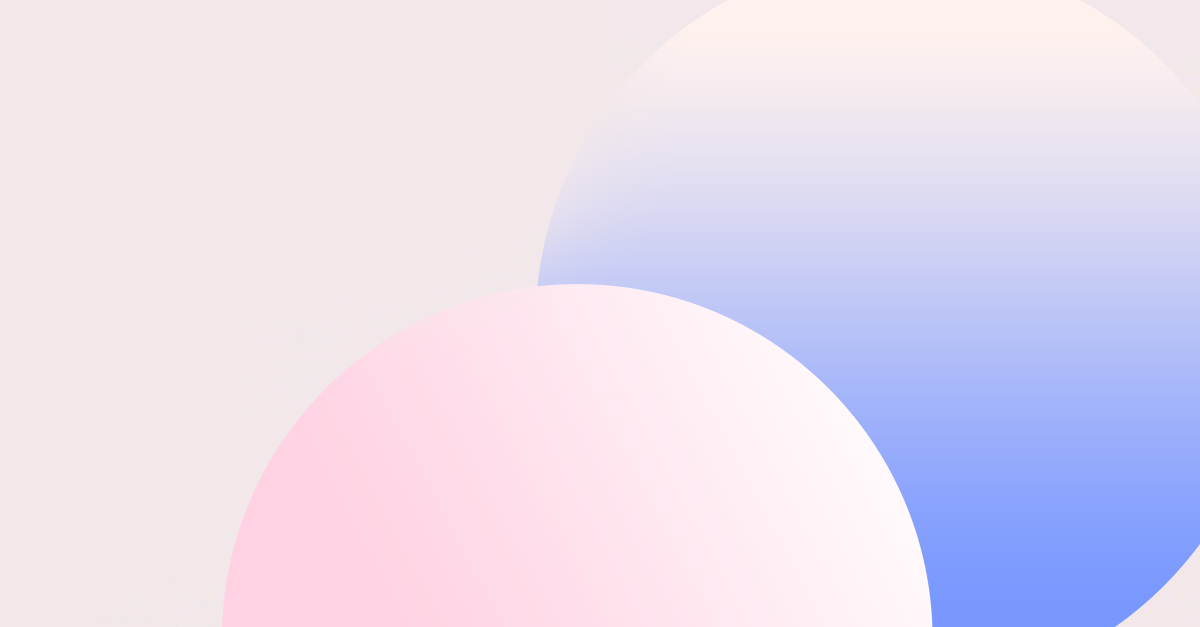
AI foundation models for audio understanding and generation
AI foundation models for audio understanding and generation, exploring standardized modeling approaches for speech recognition, synthesis, transformation, music creation, and sound effect production.
AI foundation
Audio
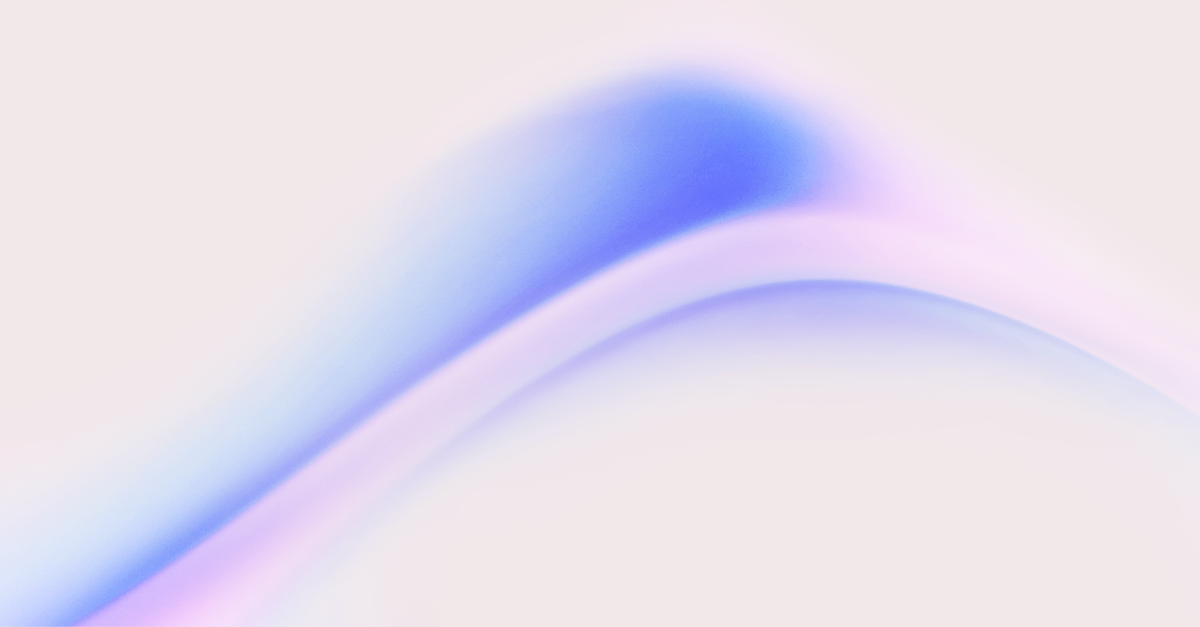
Multimodal model design and optimization
Multimodal model network architecture design and optimization, the design and refinement of diffusion models.
Multimodal
Optimization
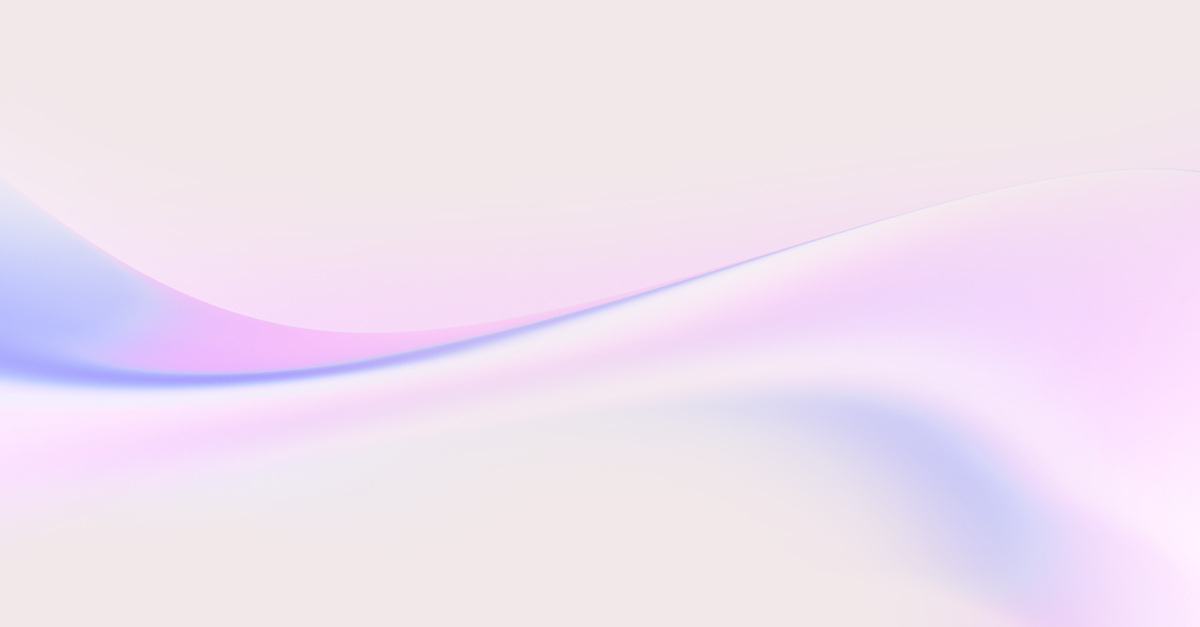
Application of reinforcement learning within environments for audio
Application of reinforcement learning within multimodal large model environments for speech/audio, and the design and enhancement of RL system solutions.
Reinforcement learning
Application
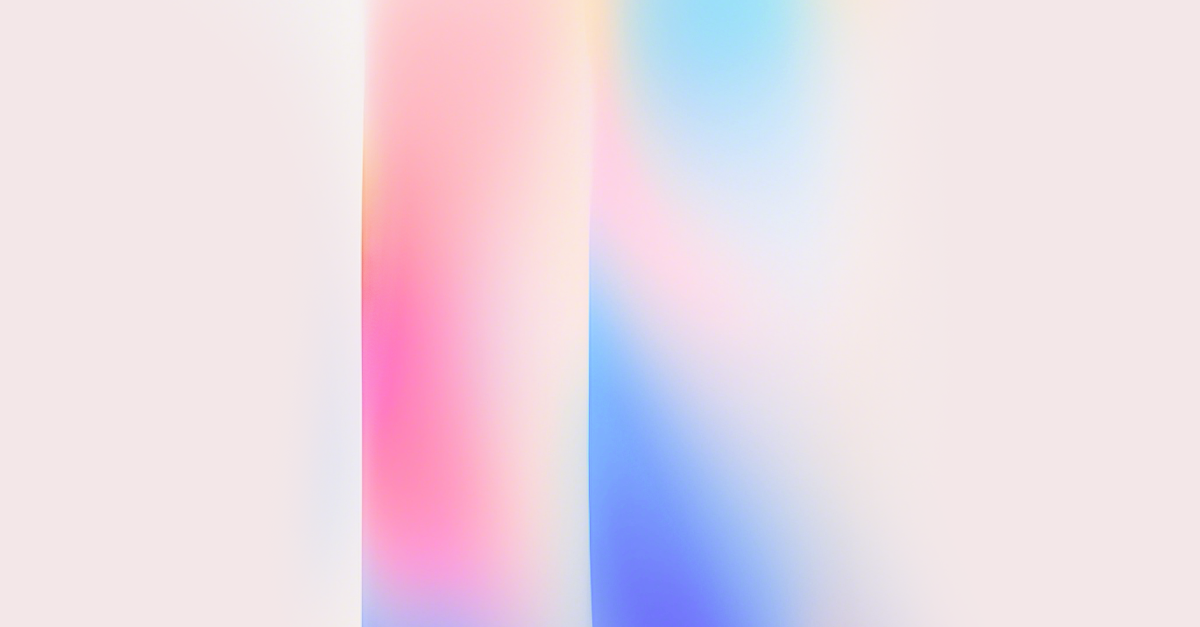
Large-scale distributed training and inference systems
Explore the development of efficient, large-scale distributed training and inference systems.
Large-scale
System
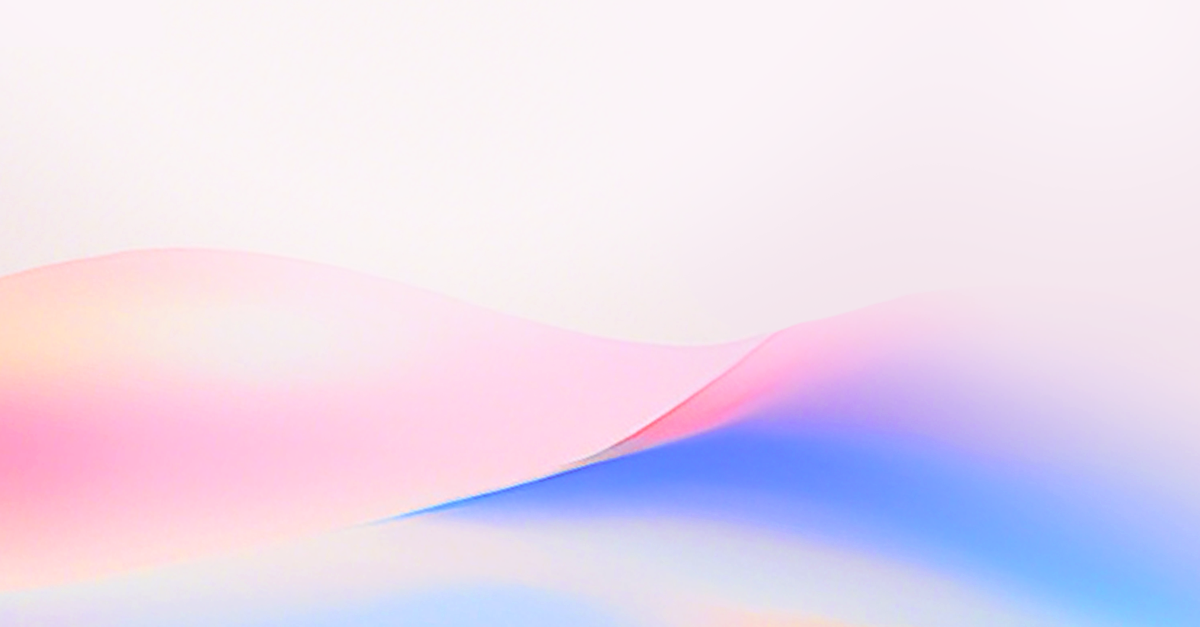
Development of machine learning platforms within environments for speech
Development of robust, scalable, and distributed machine learning platforms, aimed at facilitating the production and rapid iteration of speech/audio-related algorithms.
Machine learning
Audio
Selected Papers
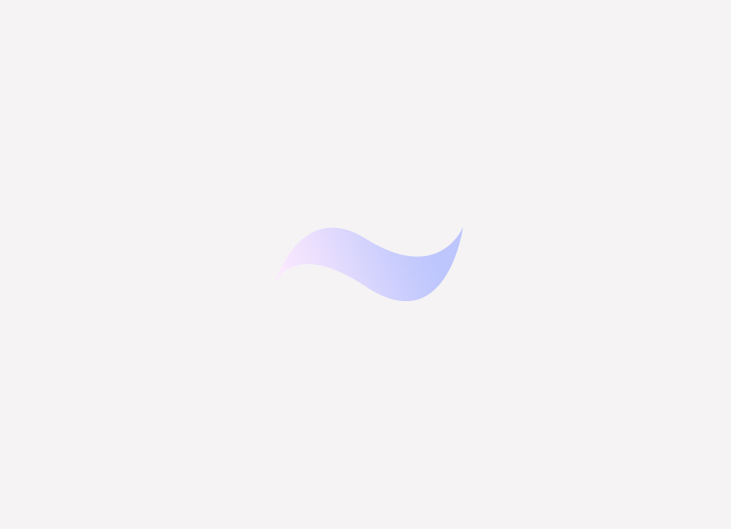
Sep 13, 2024
Seed-Music: A Unified Framework for High Quality and Controlled Music Generation
We introduce Seed-Music, a suite of music generation systems capable of producing high-quality music with fine-grained style control. Our unified framework leverages both auto-regressive language modeling and diffusion approaches to support two key music creation workflows: controlled music generation and postproduction editing. For controlled music generation, our system enables vocal music generation with performance controls from multi-modal inputs, including style descriptions, audio references, musical scores, and voice prompts. For postproduction editing, it offers interactive tools for editing lyrics and vocal melodies directly in the generated audio.
We encourage readers to listen to demo audio examples at https://team.doubao.com/seed-music.
Ye Bai, Haonan Chen, Jitong Chen, Zhuo Chen, Yi Deng, Xiaohong Dong, Lamtharn Hantrakul, Weituo Hao, Qingqing Huang, Zhongyi Huang, Dongya Jia, Feihu La, Duc Le, Bochen Li, Chumin Li, Hui Li, Xingxing Li, Shouda Liu, Wei-Tsung Lu, Yiqing Lu, Andrew Shaw, Janne Spijkervet, Yakun Sun, Bo Wang, Ju-Chiang Wang, Yuping Wang, Yuxuan Wang, Ling Xu, Yifeng Yang, Chao Yao, Shuo Zhang, Yang Zhang, Yilin Zhang, Hang Zhao, Ziyi Zhao, Dejian Zhong, Shicen Zhou, Pei Zou
Speech&Audio
2024.09.13
Seed-Music: A Unified Framework for High Quality and Controlled Music Generation
Ye Bai, Haonan Chen, Jitong Chen, Zhuo Chen, Yi Deng, Xiaohong Dong, Lamtharn Hantrakul, Weituo Hao, Qingqing Huang, Zhongyi Huang, Dongya Jia, Feihu La, Duc Le, Bochen Li, Chumin Li, Hui Li, Xingxing Li, Shouda Liu, Wei-Tsung Lu, Yiqing Lu, Andrew Shaw, Janne Spijkervet, Yakun Sun, Bo Wang, Ju-Chiang Wang, Yuping Wang, Yuxuan Wang, Ling Xu, Yifeng Yang, Chao Yao, Shuo Zhang, Yang Zhang, Yilin Zhang, Hang Zhao, Ziyi Zhao, Dejian Zhong, Shicen Zhou, Pei Zou
Speech
Speech&Audio
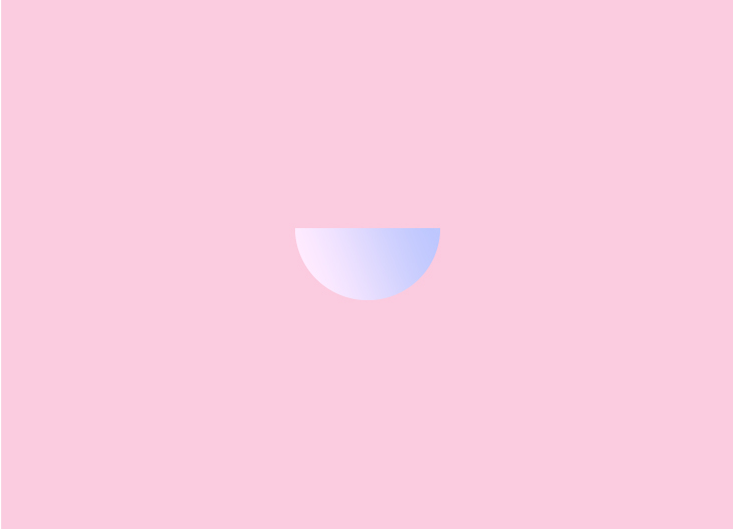
Jul 10, 2024
Seed-ASR: Understanding Diverse Speech and Contexts with LLM-based Speech Recognition
Modern automatic speech recognition (ASR) model is required to accurately transcribe diverse speech signals (from different domains, languages, accents, etc) given the specific contextual information in various application scenarios. Classic end-to-end models fused with extra language models perform well, but mainly in data matching scenarios and are gradually approaching a bottleneck. In this work, we introduce Seed-ASR, a large language model (LLM) based speech recognition model. Seed-ASR is developed based on the framework of audio conditioned LLM (AcLLM), leveraging the capabilities of LLMs by inputting continuous speech representations together with contextual information into the LLM. Through stage-wise large-scale training and the elicitation of context-aware capabilities in LLM, Seed-ASR demonstrates significant improvement over end-to-end models on comprehensive evaluation sets, including multiple domains, accents/dialects and languages. Additionally, Seed-ASR can be further deployed to support specific needs in various scenarios without requiring extra language models. Compared to recently released large ASR models, Seed-ASR achieves 10%-40% reduction in word (or character, for Chinese) error rates on Chinese and English public test sets, further demonstrating its powerful performance.
Ye Bai, Jingping Chen, Jitong Chen, Wei Chen, Zhuo Chen, Chuang Ding, Linhao Dong, Qianqian Dong, Yujiao Du, Kepan Gao, Lu Gao, Yi Guo, Minglun Han, Ting Han, Wenchao Hu, Xinying Hu, Yuxiang Hu, Deyu Hua, Lu Huang, Mingkun Huang, Youjia Huang, Jishuo Jin, Fanliu Kong, Zongwei Lan, Tianyu Li, Xiaoyang Li, Zeyang Li, Zehua Lin, Rui Liu, Shouda Liu, Lu Lu, Yizhou Lu, Jingting Ma, Shengtao Ma, Yulin Pei, Chen Shen, Tian Tan, Xiaogang Tian, Ming Tu, Bo Wang, Hao Wang, Yuping Wang, Yuxuan Wang, Hanzhang Xia, Rui Xia, Shuangyi Xie, Hongmin Xu, Meng Yang, Bihong Zhang, Jun Zhang, Wanyi Zhang, Yang Zhang, Yawei Zhang, Yijie Zheng, Ming Zou
Speech&Audio
2024.07.10
Seed-ASR: Understanding Diverse Speech and Contexts with LLM-based Speech Recognition
Ye Bai, Jingping Chen, Jitong Chen, Wei Chen, Zhuo Chen, Chuang Ding, Linhao Dong, Qianqian Dong, Yujiao Du, Kepan Gao, Lu Gao, Yi Guo, Minglun Han, Ting Han, Wenchao Hu, Xinying Hu, Yuxiang Hu, Deyu Hua, Lu Huang, Mingkun Huang, Youjia Huang, Jishuo Jin, Fanliu Kong, Zongwei Lan, Tianyu Li, Xiaoyang Li, Zeyang Li, Zehua Lin, Rui Liu, Shouda Liu, Lu Lu, Yizhou Lu, Jingting Ma, Shengtao Ma, Yulin Pei, Chen Shen, Tian Tan, Xiaogang Tian, Ming Tu, Bo Wang, Hao Wang, Yuping Wang, Yuxuan Wang, Hanzhang Xia, Rui Xia, Shuangyi Xie, Hongmin Xu, Meng Yang, Bihong Zhang, Jun Zhang, Wanyi Zhang, Yang Zhang, Yawei Zhang, Yijie Zheng, Ming Zou
Speech
Speech&Audio
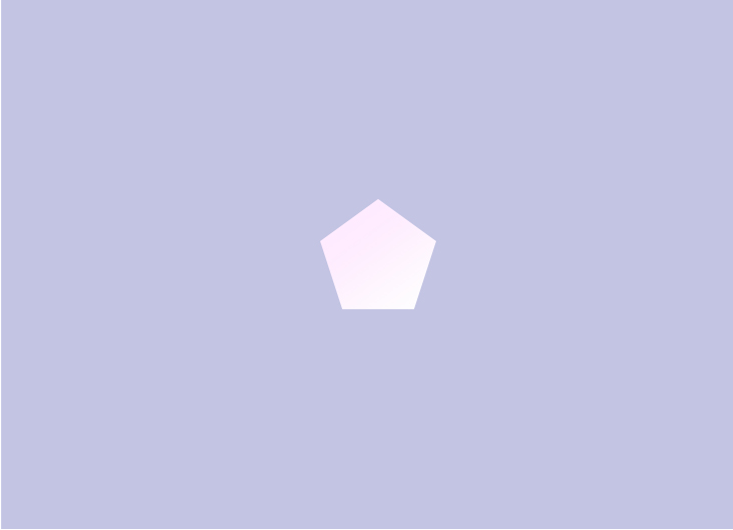
Jun 04, 2024
Seed-TTS: A Family of High-Quality Versatile Speech Generation Models
We introduce Seed-TTS, a family of large-scale autoregressive text-to-speech
(TTS) models capable of generating speech that is virtually indistinguishable from
human speech. Seed-TTS serves as a foundation model for speech generation and
excels in speech in-context learning, achieving performance in speaker similarity
and naturalness that matches ground truth human speech in both objective and
subjective evaluations. With fine-tuning, we achieve even higher subjective scores
across these metrics. Seed-TTS offers superior controllability over various speech
attributes such as emotion and is capable of generating highly expressive and diverse
speech for speakers in the wild. Furthermore, we propose a self-distillation method
for speech factorization, as well as a reinforcement learning approach to enhance
model robustness, speaker similarity, and controllability. We additionally present a
non-autoregressive (NAR) variant of the Seed-TTS model, named Seed-TTSDiT,
which utilizes a fully diffusion-based architecture. Unlike previous NAR-based
TTS systems, Seed-TTSDiT does not depend on pre-estimated phoneme durations
and performs speech generation through end-to-end processing. We demonstrate
that this variant achieves comparable performance to the language model-based
variant and showcase its effectiveness in speech editing. We encourage readers
to listen to demos at https://bytedancespeech.github.io/seedtts_tech_report/
Philip Anastassiou, Jiawei Chen, Jitong Chen, Yuanzhe Chen, Zhuo Chen, Ziyi Chen, Jian Cong, Lelai Deng, Chuang Ding, Lu Gao, Mingqing Gong, Peisong Huang, Qingqing Huang, Zhiying Huang, Yuanyuan Huo, Dongya Jia, Chumin Li, Feiya Li, Hui Li, Jiaxin Li, Xiaoyang Li, Xingxing Li, Lin Liu, Shouda Liu, Sichao Liu, Xudong Liu, Yuchen Liu, Zhengxi Liu, Lu Lu, Junjie Pan, Xin Wang, Yuping Wang, Yuxuan Wang, Zhen Wei, Jian Wu, Chao Yao, Yifeng Yang, Yuanhao Yi, Junteng Zhang, Qidi Zhang, Shuo Zhang, Wenjie Zhang, Yang Zhang, Zilin Zhao, Dejian Zhong, Xiaobin Zhuang
Speech&Audio
2024.06.04
Seed-TTS: A Family of High-Quality Versatile Speech Generation Models
Philip Anastassiou, Jiawei Chen, Jitong Chen, Yuanzhe Chen, Zhuo Chen, Ziyi Chen, Jian Cong, Lelai Deng, Chuang Ding, Lu Gao, Mingqing Gong, Peisong Huang, Qingqing Huang, Zhiying Huang, Yuanyuan Huo, Dongya Jia, Chumin Li, Feiya Li, Hui Li, Jiaxin Li, Xiaoyang Li, Xingxing Li, Lin Liu, Shouda Liu, Sichao Liu, Xudong Liu, Yuchen Liu, Zhengxi Liu, Lu Lu, Junjie Pan, Xin Wang, Yuping Wang, Yuxuan Wang, Zhen Wei, Jian Wu, Chao Yao, Yifeng Yang, Yuanhao Yi, Junteng Zhang, Qidi Zhang, Shuo Zhang, Wenjie Zhang, Yang Zhang, Zilin Zhao, Dejian Zhong, Xiaobin Zhuang
Speech
Speech&Audio
Learn More
Technical capability demonstration
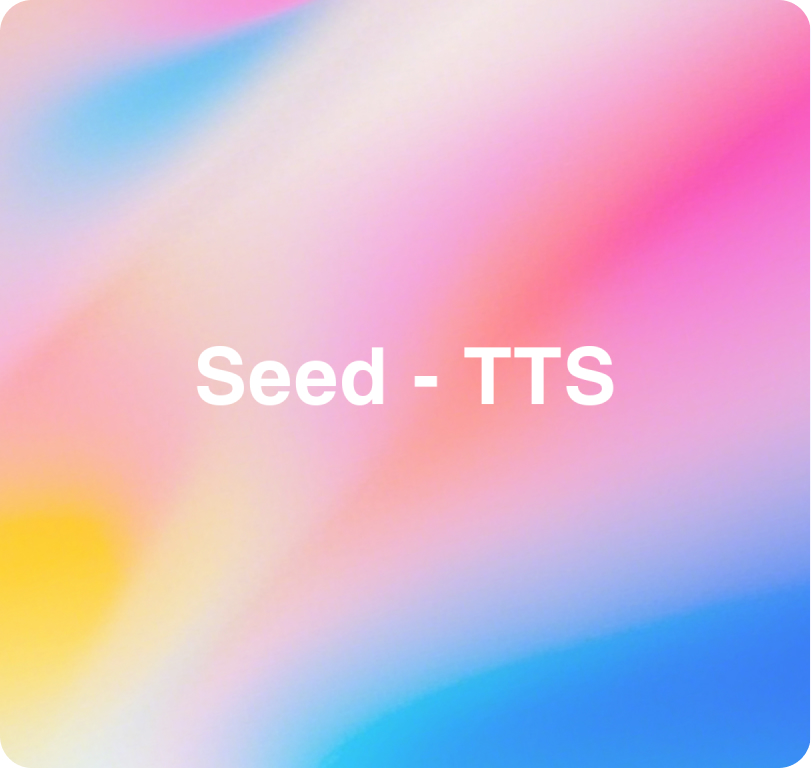
Seed-TTS
Doubao · Speech Generation Model shows superior context comprehension and naturalness, capable of deeply understanding narratives and character roles, expressing emotions accurately, and maintaining specific pronunciation habits such as swallowing sounds and accents, closely mimicking human vocal quality.
Speech
Generation
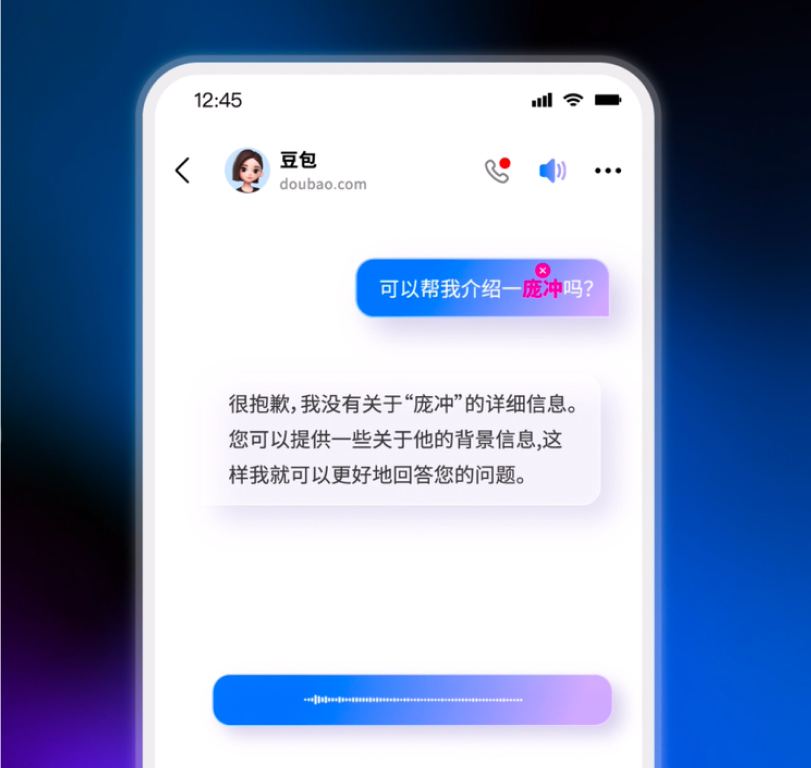
Seed-ASR
Doubao · Speech Recognition Model leverages more advanced context-aware capabilities to produce more precise recognition outcomes and support the recognition of multiple Chinese dialects, including Mandarin, Cantonese, Shanghainese, Sichuanese, Xi'an dialect, and Minnan, within a single model.
Speech
Recognition
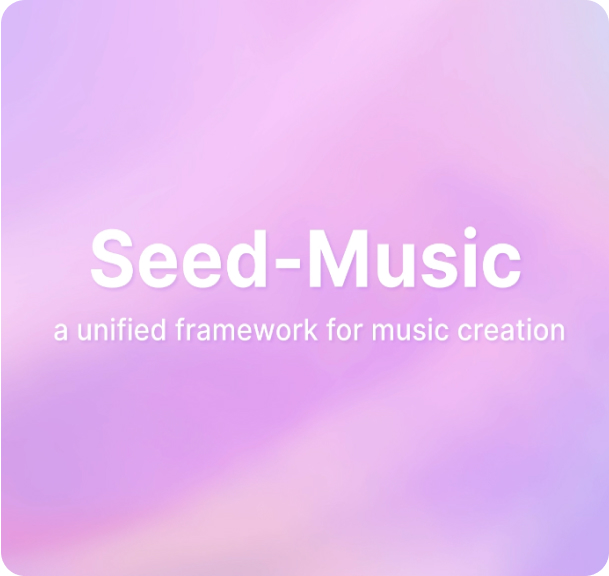
Seed-Music
Seed-Music is a collection of music generation models with flexible control capabilities, offering four core functions: controllable music generation, score-to-music conversion, lyric and music editing, and zero-sample vocal cloning. By smartly merging the benefits of language and diffusion models into the composition process, Music makes crafting songs accessible to everyone.
Music
Generation
Featured Jobs
Research Scientist, Multimodality
Research Scientist, Foundation Model, Music Intelligence
Research Scientist in Foundation Model, Speech & Audio Generation - 2025 Start (PhD)
Research Scientist in Foundation Model, Music - 2025 Start (PhD)
Student Researcher (Doubao (Seed) - Foundation Model - Speech Understanding) - 2025 Start (PhD)
Student Researcher (Doubao (Seed) - Music Foundation Model) - 2025 Start (PhD)