Vision
豆包大模型视觉团队致力于视觉生成的基础模型、多模态生成模型、以及基于生成式 AI 视觉基础问题的前沿科研和应用研发
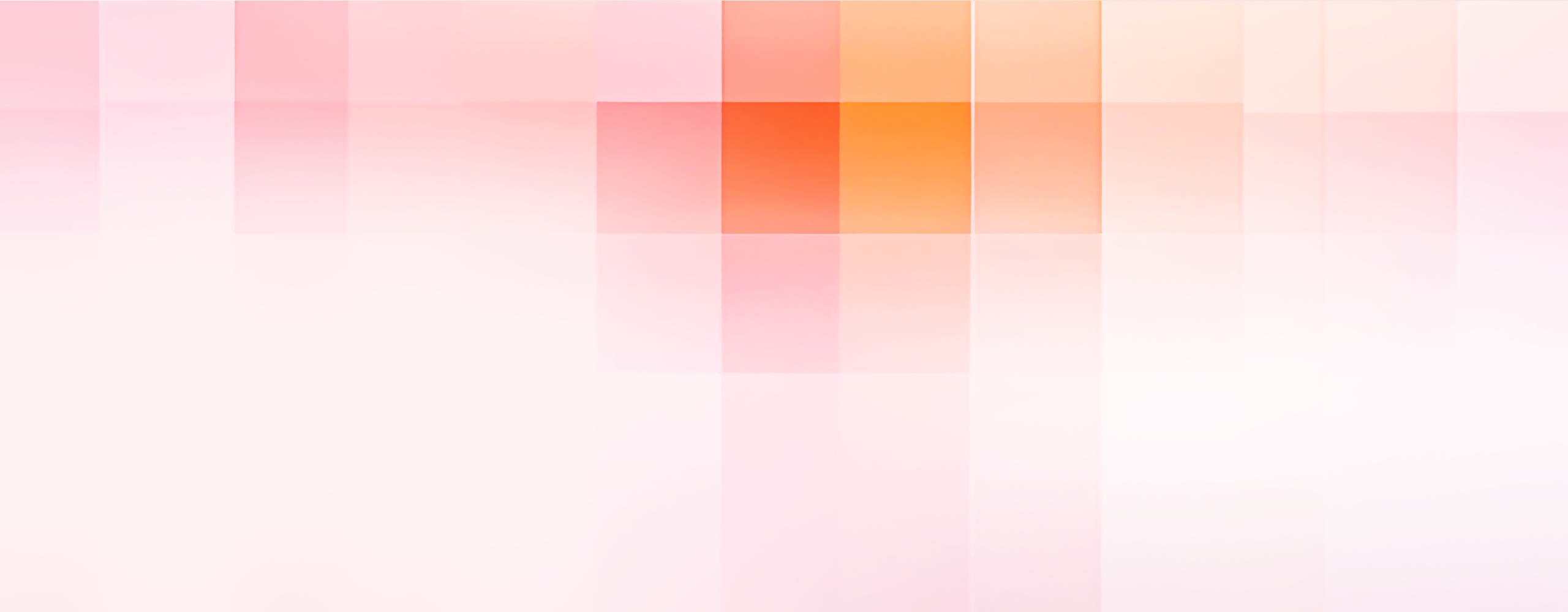
研究领域
研究方向
团队专注于视觉生成模型,多模态架构,以及人工智能视觉相关领域的技术研究
探索课题
包括 AIGC、扩散模型、自回归模型、多模态模型、3D/4D 生成、视觉自监督学习、模型优化加速等
课题方向
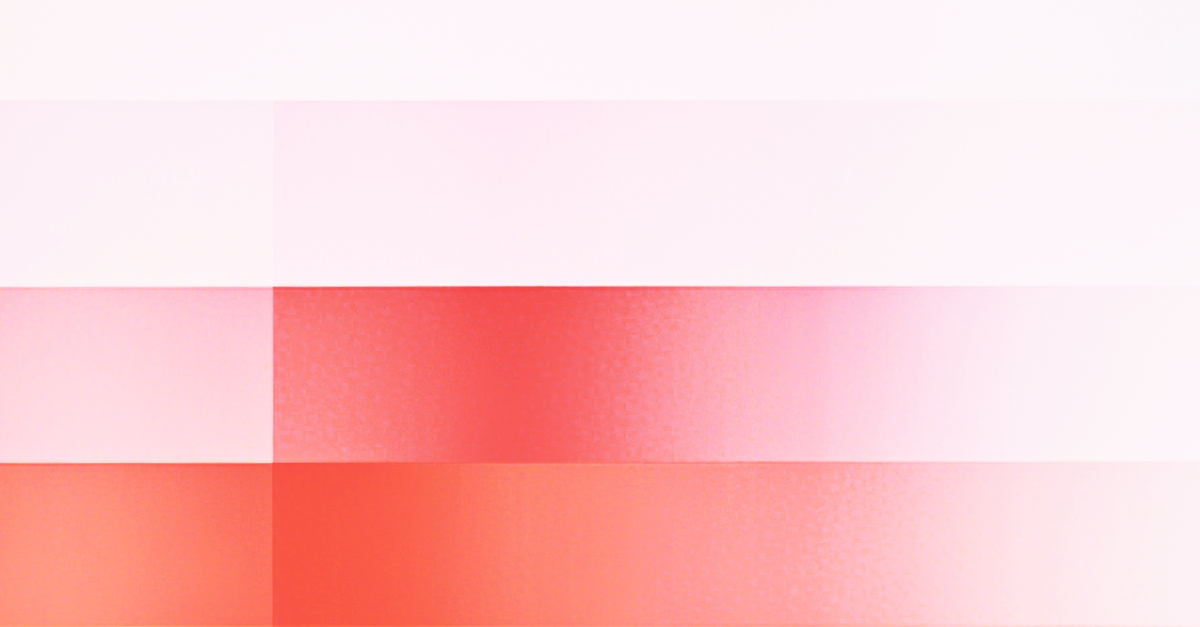
视觉生成基础模型
研发视觉生成(图像和视频)的基座模型,提供视觉生成高交互性和高可控性,理解视频中的视觉规律,探索基于生成基座模型的各种视觉任务
Multimodal
Diffusion Model
Auto Regression Model
Foundation
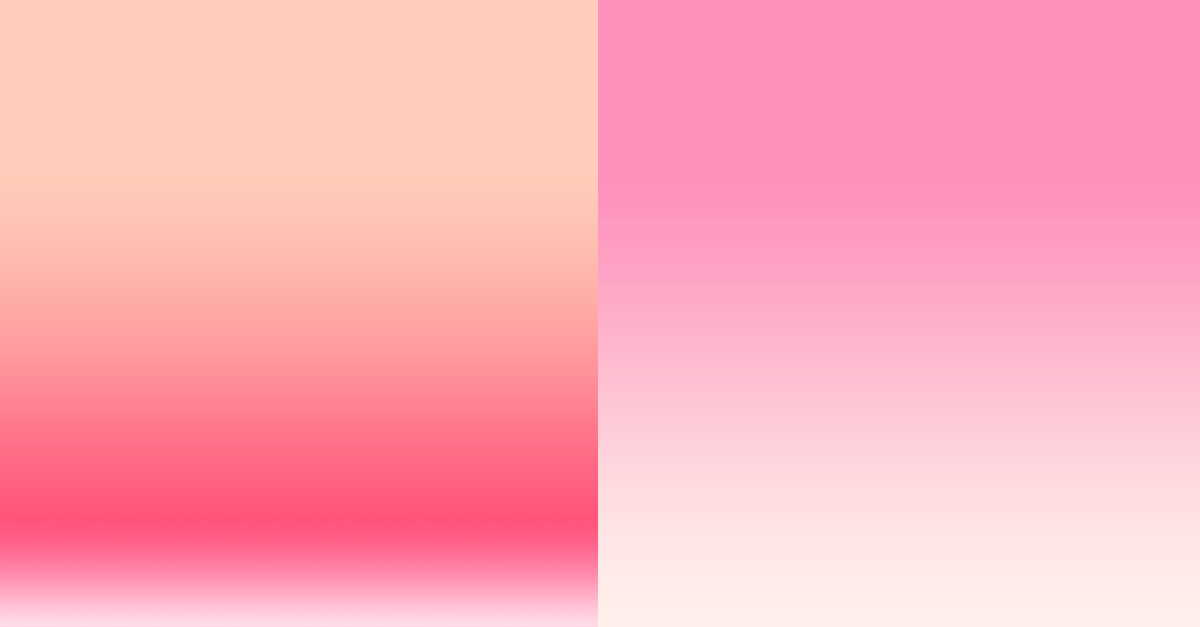
多模态生成模型
融合多种模态的统一生成模型,生成和理解联合建模,支持多模态的交织生成和同时生成(E.g. 数字人),提升生成模型上下文能力和一致性
Multimodel
Diffusion Model
Auto Regression Model
Foundation
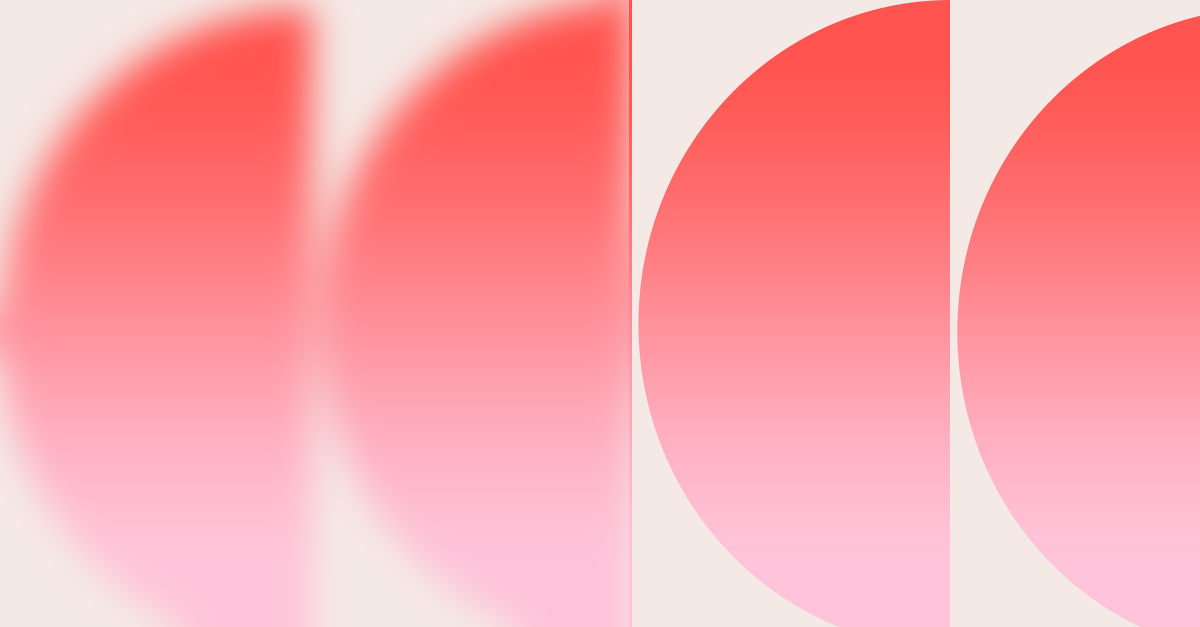
3D/4D 生成模型
3D/4D 生成基础模型,从视频数据和 3D 数据学习视觉世界知识,理解物理世界 3D 空间和物理规律,构建视觉的空间智能和世界模型,探索基于生成模型的物理和渲染引擎
3D
4D
World Model
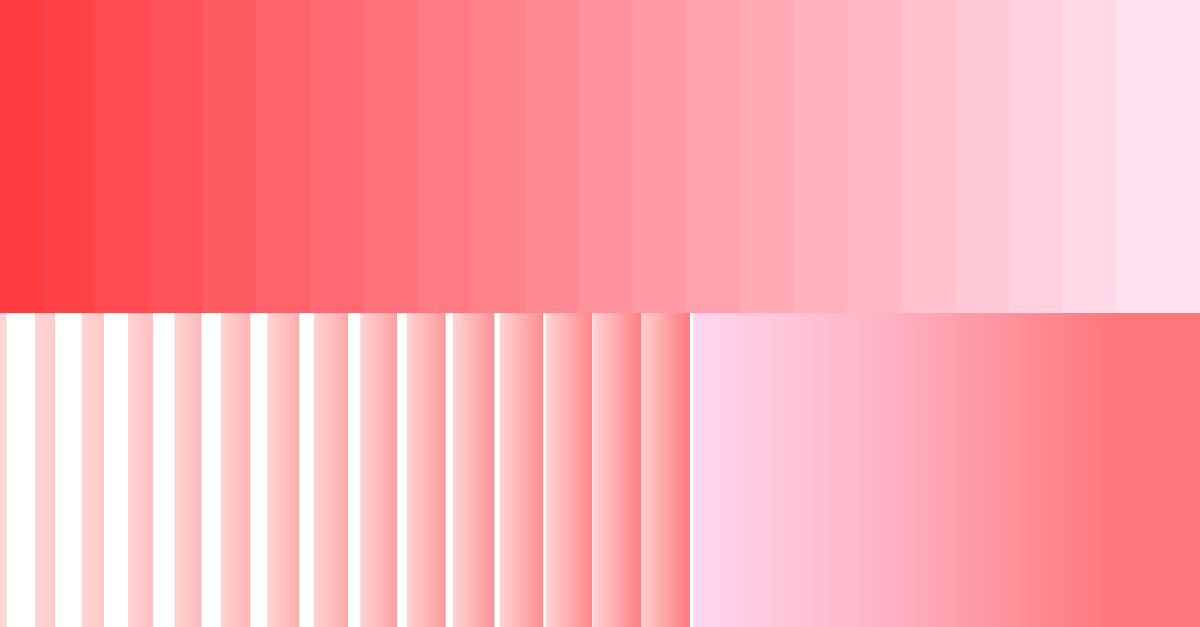
多模态模型设计和优化
多模态模型网络架构设计和优化、扩散模型的优化、高效的大规模分布式训练和推理、模型加速和优化
Multimodal
Optimization
Distillation
Quantization
精选论文
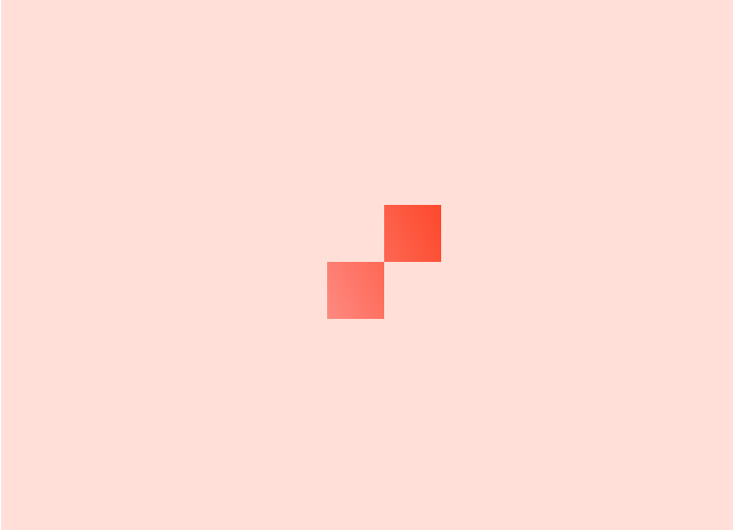
2024.11.11
SeedEdit: Align Image Re-Generation to Image Editing
We introduce SeedEdit, a diffusion model that is able to revise a given image with any text prompt. In our perspective, the key to such a task is to obtain an optimal balance between maintaining the original image, i.e. image reconstruction, and generating a new image, i.e. image re-generation. To this end, we start from a weak generator (text-to-image model) that creates diverse pairs between such two directions and gradually align it into a strong image editor that well balances between the two tasks. SeedEdit can achieve more diverse and stable editing capability over prior image editing methods, enabling sequential revision over images generated by diffusion models.
Yichun Shi, Peng Wang, Weilin Huang
Computer Vision
2024.11.11
SeedEdit: Align Image Re-Generation to Image Editing
Yichun Shi, Peng Wang, Weilin Huang
Vision
Computer Vision
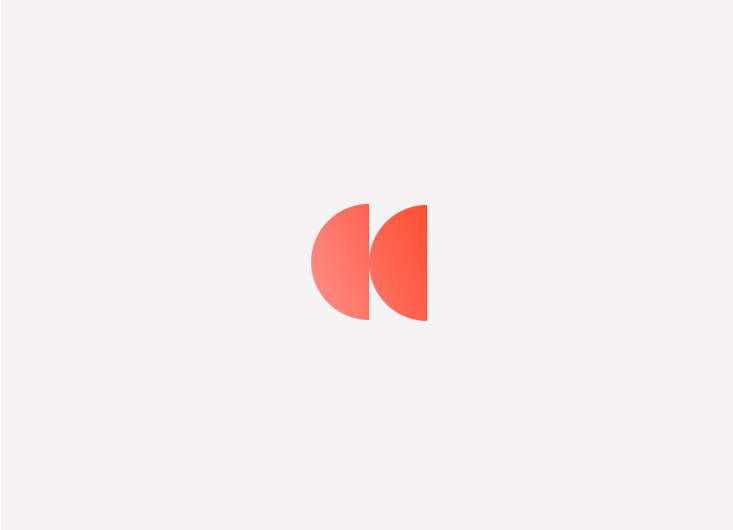
2024.11.04
How Far is Video Generation from World Model: A Physical Law Perspective
OpenAI's Sora highlights the potential of video generation for developing world models that adhere to fundamental physical laws. However, the ability of video generation models to discover such laws purely from visual data without human priors can be questioned. A world model learning the true law should give predictions robust to nuances and correctly extrapolate on unseen scenarios. In this work, we evaluate across three key scenarios: in-distribution, out-of-distribution, and combinatorial generalization. We developed a 2D simulation testbed for object movement and collisions to generate videos deterministically governed by one or more classical mechanics laws. This provides an unlimited supply of data for large-scale experimentation and enables quantitative evaluation of whether the generated videos adhere to physical laws. We trained diffusion-based video generation models to predict object movements based on initial frames. Our scaling experiments show perfect generalization within the distribution, measurable scaling behavior for combinatorial generalization, but failure in out-of-distribution scenarios. Further experiments reveal two key insights about the generalization mechanisms of these models: (1) the models fail to abstract general physical rules and instead exhibit "case-based" generalization behavior, i.e., mimicking the closest training example; (2) when generalizing to new cases, models are observed to prioritize different factors when referencing training data: color > size > velocity > shape. Our study suggests that scaling alone is insufficient for video generation models to uncover fundamental physical laws, despite its role in Sora's broader success. See our project page at https://phyworld.github.io/
Bingyi Kang, Yang Yue, Rui Lu, Zhijie Lin, Yang Zhao, Kaixin Wang, Gao Huang, Jiashi Feng
Computer Vision
2024.11.04
How Far is Video Generation from World Model: A Physical Law Perspective
Bingyi Kang, Yang Yue, Rui Lu, Zhijie Lin, Yang Zhao, Kaixin Wang, Gao Huang, Jiashi Feng
Vision
Computer Vision
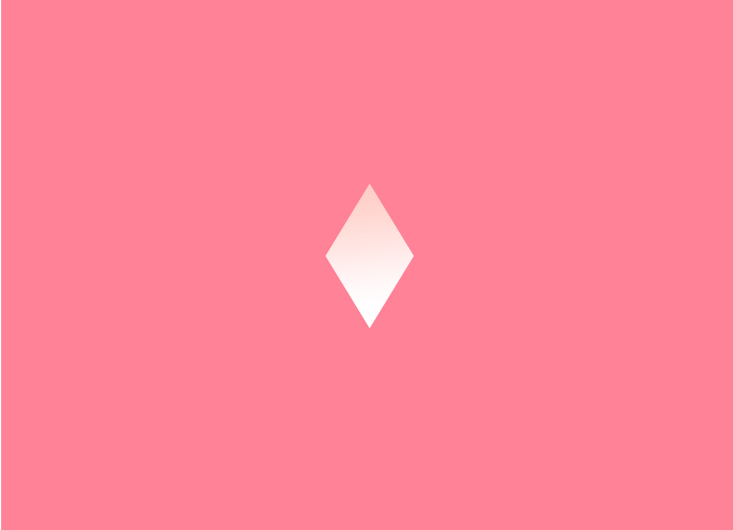
2024.04.21
Hyper-SD: Trajectory Segmented Consistency Model for Efficient Image Synthesis
Recently, a series of diffusion-aware distillation algorithms have emerged to alleviate the computational overhead associated with the multi-step inference process of Diffusion Models (DMs). Current distillation techniques often dichotomize into two distinct aspects: i) ODE Trajectory Preservation; and ii) ODE Trajectory Reformulation. However, these approaches suffer from severe performance degradation or domain shifts. To address these limitations, we propose Hyper-SD, a novel framework that synergistically amalgamates the advantages of ODE Trajectory Preservation and Reformulation, while maintaining near-lossless performance during step compression. Firstly, we introduce Trajectory Segmented Consistency Distillation to progressively perform consistent distillation within pre-defined time-step segments, which facilitates the preservation of the original ODE trajectory from a higher-order perspective. Secondly, we incorporate human feedback learning to boost the performance of the model in a low-step regime and mitigate the performance loss incurred by the distillation process. Thirdly, we integrate score distillation to further improve the low-step generation capability of the model and offer the first attempt to leverage a unified LoRA to support the inference process at all steps. Extensive experiments and user studies demonstrate that Hyper-SD achieves SOTA performance from 1 to 8 inference steps for both SDXL and SD1.5. For example, Hyper-SDXL surpasses SDXL-Lightning by +0.68 in CLIP Score and +0.51 in Aes Score in the 1-step inference.
Yuxi Ren, Xin Xia, Yanzuo Lu, Jiacheng Zhang, Jie Wu, Pan Xie, Xing Wang, Xuefeng Xiao
Computer Vision
2024.04.21
Hyper-SD: Trajectory Segmented Consistency Model for Efficient Image Synthesis
Yuxi Ren, Xin Xia, Yanzuo Lu, Jiacheng Zhang, Jie Wu, Pan Xie, Xing Wang, Xuefeng Xiao
Vision
Computer Vision
查看更多论文
技术应用
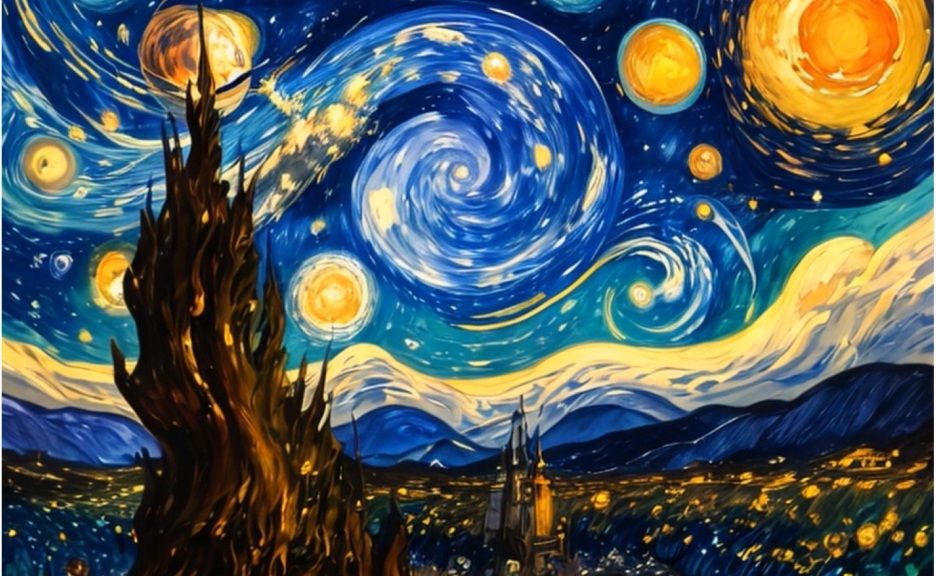
豆包·文生图模型
豆包·文生图模型现已应用于抖音、剪映、豆包、星绘等产品。在豆包 App 中输入提示词,即可生成兼具光影明暗、氛围色彩和人物美感的高质量图像,同时支持中英文双语输入,对复杂 prompt 的理解同样精准。
Text-to-Image
Model
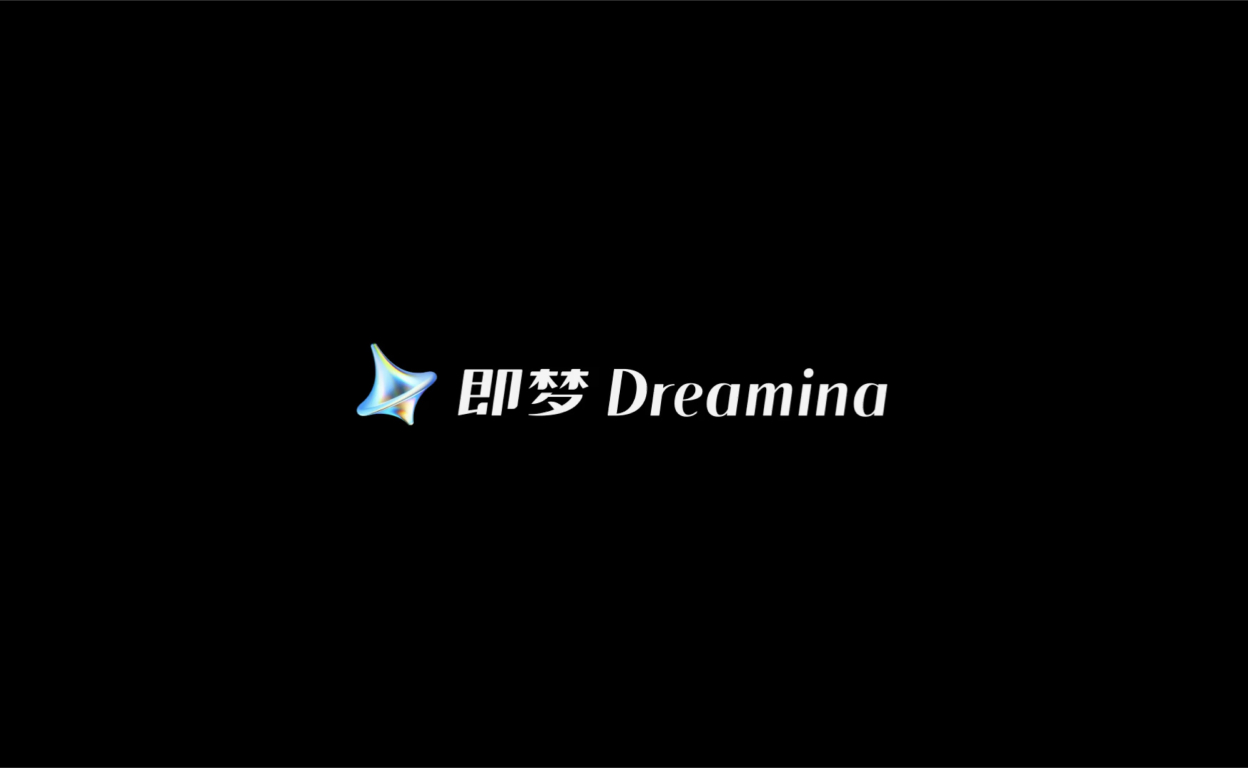
即梦
即梦是一款由字节跳动自主研发的 AI 创作产品,支持通过自然语言及图片输入,生成高质量的图像及视频。平台提供智能画布、故事创作模式及各种 AI 编辑能力,为用户的创作提效。
AI-powered
Creative